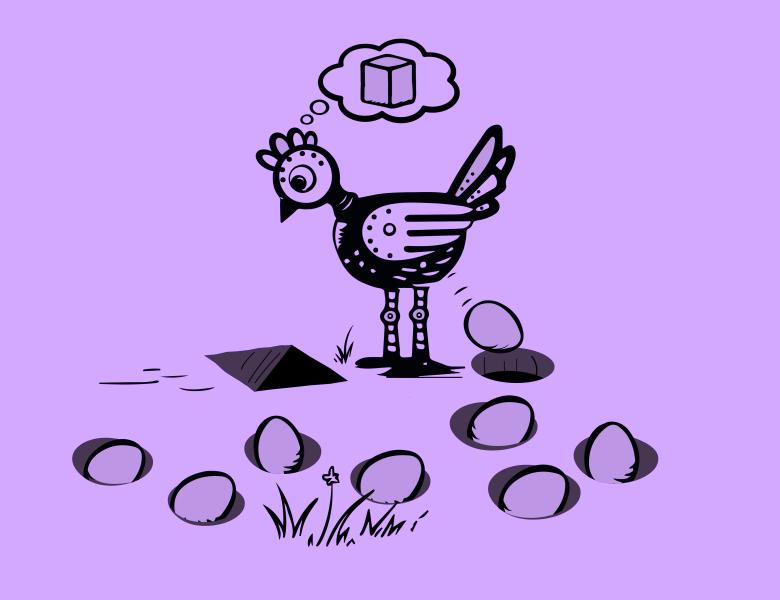
About
Generalization, broadly construed, is the ability of machine learning methods to perform well in scenarios outside their training data.
Despite being a well-developed field with a rich history, contemporary phenomena — in particular, those arising from deep learning, most specifically large image and language models — are well beyond our current mathematical tool kit and vocabulary. It is not merely that analyses are too loose to be effective; rather, the settings have drastically evolved from the standard statistical setting of similar training and testing data, as the following examples illuminate: self-driving cars may need to navigate unfamiliar and even private or inaccessible roads; image generation software is expected to provide compelling images from essentially arbitrary input strings, with human operators indeed enjoying breaking the training data mold; AlphaFold and related software make protein predictions for species unrelated to those in their training set. The list goes on, without even scratching the surface of large language models and algorithmic tasks.
This program's goal is to bring together remote and local researchers, in both academia and industry, as well as across mathematical and applied disciplines, with the common goals of (a) organizing and crystallizing gaps between the theory and practice of generalization, and (b) sparking collaboration toward a concerted effort to close these gaps.