
We are heartbroken by the loss of Luca Trevisan, who served as senior scientist at the Institute from 2014 to 2019.
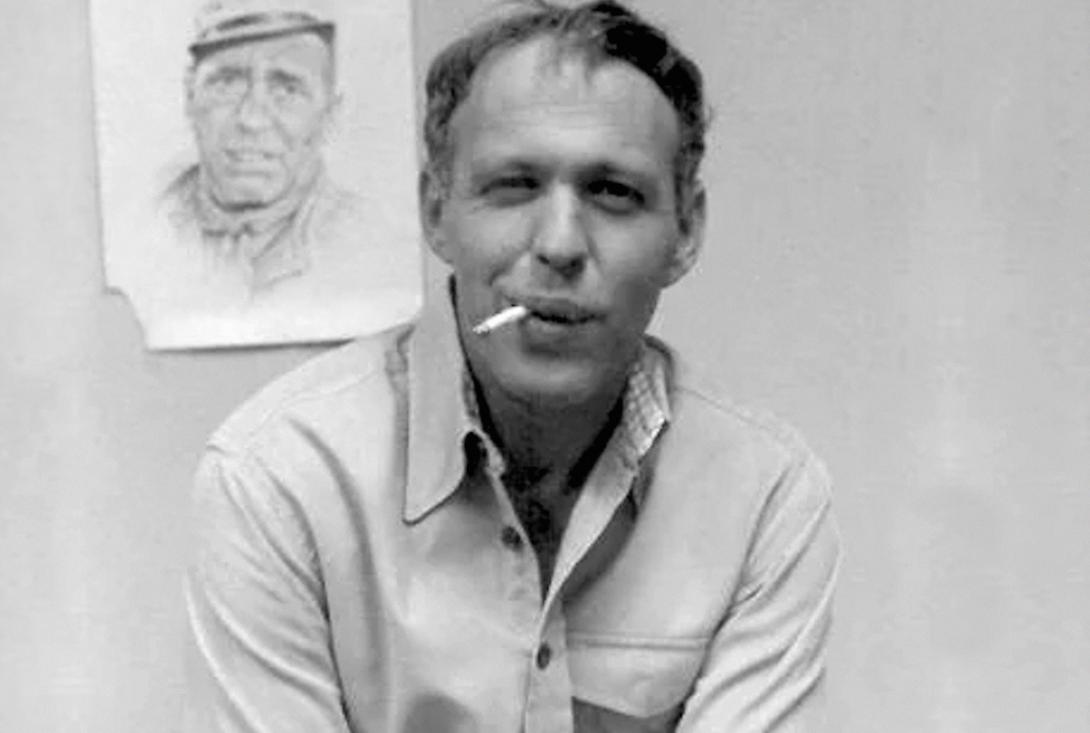
Appreciation and memories of Jim Simons (1938–2024), from the Simons Institute community. Featuring contributions from Avi Wigderson, Dick Karp, Shafi...

Simons Institute Senior Scientist Venkatesan Guruswami, along with Bingkai Lin, Yican Sun, and Berkeley theory graduate students Xuandi Ren and Kewen...

We are heartbroken by the loss of Luca Trevisan, who served as senior scientist at the Institute from 2014 to 2019.

Appreciation and memories of Jim Simons (1938–2024), from the Simons Institute community. Featuring contributions from Avi Wigderson, Dick Karp, Shafi Goldwasser, Bob Birgeneau, Alistair Sinclair, Venkatesan Guruswami, Nikhil Srivastava, Michael Sipser, Tim Roughgarden, Vijay Vazirani, Lin Lin, Virginia Vassilevska Williams, Omer Reingold, Bonnie Berger, Ravindran Kannan, Aviv Regev, Subhash Khot, Noga Alon, Rocco Servedio, Barbara Simons, Bin Yu, Amit Sahai, Shang-Hua Teng, Dan Boneh, Shachar Lovett, Greg Valiant, and Ronitt Rubinfeld.
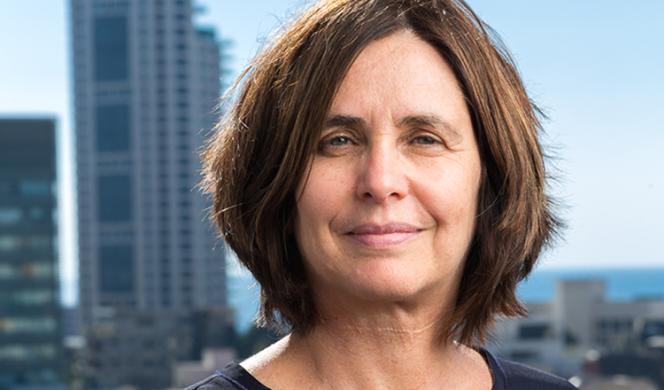
Dear friends,
Jim Simons, the larger-than-life mathematician-entrepreneur-philanthropist to whom the Simons Institute for the Theory of Computing owes its existence, passed away last month. Many of those in our research community have been reflecting on Jim’s wisdom, generosity, and humanity over the last few weeks. I share some of those remembrances with you in this issue of the newsletter, along with the obituary published by Berkeley News. We bid farewell to a great man.
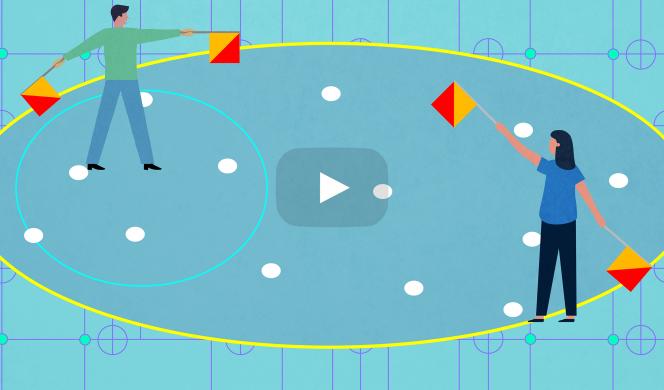
Sivakanth Gopi (Microsoft Research) describes recent developments in the theory of higher-order MDS codes and their surprising connections to central coding-theoretic concepts like list decoding and maximal recoverability.

In this presentation from the Workshop in Honor of Michael Ben-Or, John Preskill (Caltech) gives a historical tour of some important developments by Ben-Or and others in quantum fault tolerance.
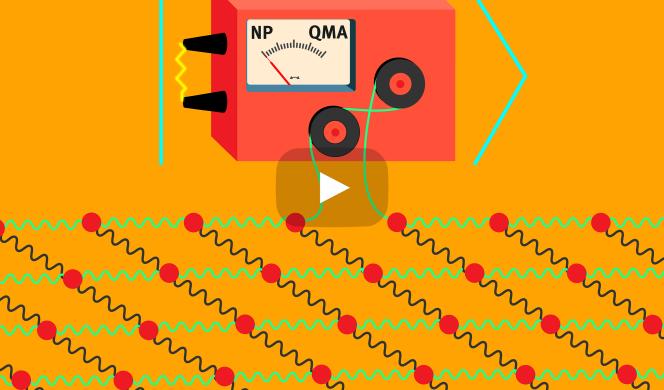
We share here one of our favorite talks from the workshop on Near-Term Quantum Computers: Fault Tolerance + Benchmarking + Quantum Advantage + Quantum Algorithms. Yunchao Liu (UC Berkeley) presents joint work with Zeph Landau (UC Berkeley).
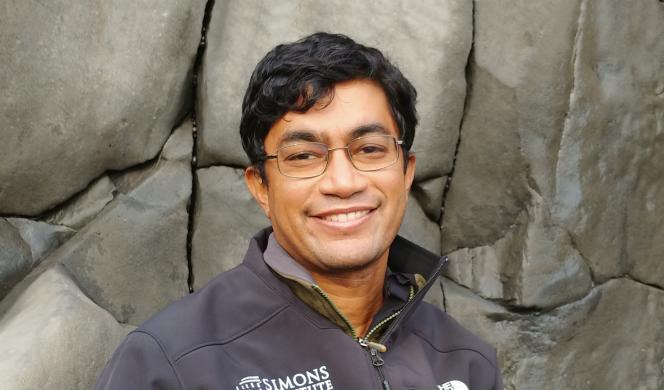
Simons Institute Senior Scientist Venkatesan Guruswami, along with Bingkai Lin, Yican Sun, and Berkeley theory graduate students Xuandi Ren and Kewen Wu, received a best paper award at the 56th ACM Symposium on Theory of Computing conference, for their paper, "Parameterized Inapproximability Hypothesis under ETH."

Dear friends,
I deeply mourn the passing of Jim Simons, the founding benefactor of the Simons Institute for the Theory of Computing, whose vision, generosity, and commitment to mathematics and the sciences have changed the face of theoretical computer science and the Berkeley campus. His legacy will be felt for generations.
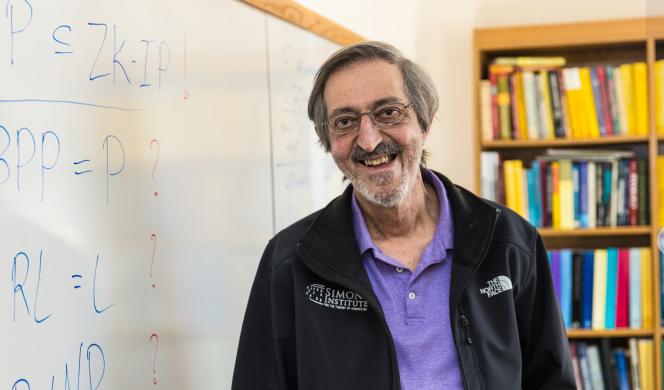
In celebration of Avi Wigderson’s receipt of the ACM A.M. Turing Award this year, we put together a playlist of Avi’s talks at the Simons Institute.
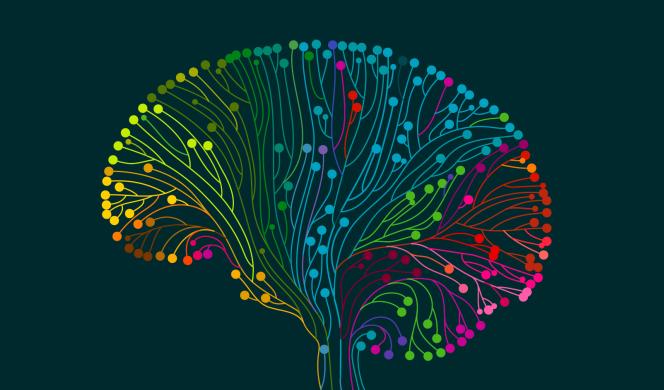
In April, the Simons Institute launched a Research Pod on Resilience in Brain, Natural, and Algorithmic Systems. The Resilience Pod will explore the topic of resilience through an interdisciplinary lens, over the course of three years.