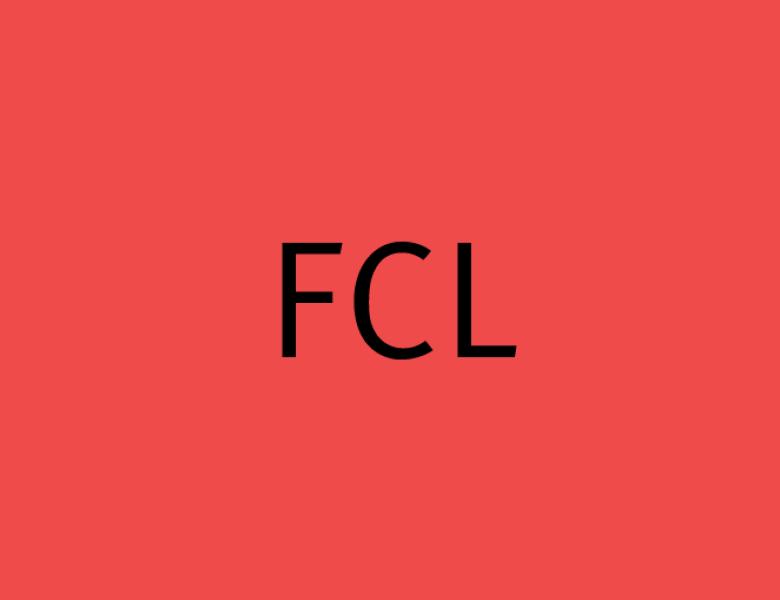
About
Modern data generation — from individuals' personal devices, in smart homes and cities, within hospitals or financial institutions — fundamentally changes data analyses from classical scenarios, where we view data as a sample from a single large underlying population. These new data modes result in heterogeneous siloed data residing in the devices or organizations that generated it. Federated learning (FL) considers collaboratively learning across heterogeneous networks (e.g., networks of mobile phones, collections of hospitals). Federated approaches aim to reduce computational costs and mitigate systemic privacy risks that stem from traditional, centralized approaches to machine learning and analytics.
More generally, machine learning pipelines are increasingly powered by data from multiple sources and stakeholders. This yields foundational new questions for machine learning and statistics. For example, how can we trade between individual and global utility? What can one learn with a single individual’s data, and can collaboration improve this? How can we ensure successful collaboration while preventing potential risks? Techniques for collaborative learning, such as FL, stand to power a new generation of ML applications by enabling coordinated, trustworthy learning among multiple parties and across diverse data sources. To do so, novel approaches must be developed to improve the accuracy and efficiency of learning across siloed data; mitigate risk and protect data privacy and ownership; and incorporate social and economic principles that incentivize data sharing and provide trustworthy cooperative learning schemes.
There has been promising initial progress in the theoretical study of federated and collaborative learning, as well as in emerging real-world applications. However, these branches have proceeded largely independently, missing opportunities for progress. This program will bring together disparate communities, all working on different aspects of federated and collaborative learning, to develop foundational tools and shape the agenda of future research.
Long-Term Participants (tentative):
Zachary Charles (Google), Mosharaf Chowdhury (University of Michigan), Rachel Cummings (Columbia University), Kate Donahue (Cornell University), John Duchi (Stanford University), Giulia Fanti (CMU), Vitaly Feldman (Apple), Nika Haghtalab (UC Berkeley), Peter Kairouz (Google), Sai Praneeth Karimireddy (UC Berkeley), Anastasia Koloskova (EPFL), Sanmi Koyejo (Stanford University), Tian Li (CMU), Katrina Ligett (Hebrew University), Audra McMillan (Apple), Peter Richtarik (KAUST), Aadirupa Saha (Apple), Adam Smith (Boston University), Virginia Smith (CMU), Nati Srebro (TTIC), Thomas Steinke (Google), Kunal Talwar (Apple), Jonathan Ullman (Northeastern University), Shanshan Wu (Google), Zheng Xu (Google)
To participate as a fellow, please apply here.
To participate as a long-term visitor, please apply here.
To participate as a Law&Society fellow, please apply here.