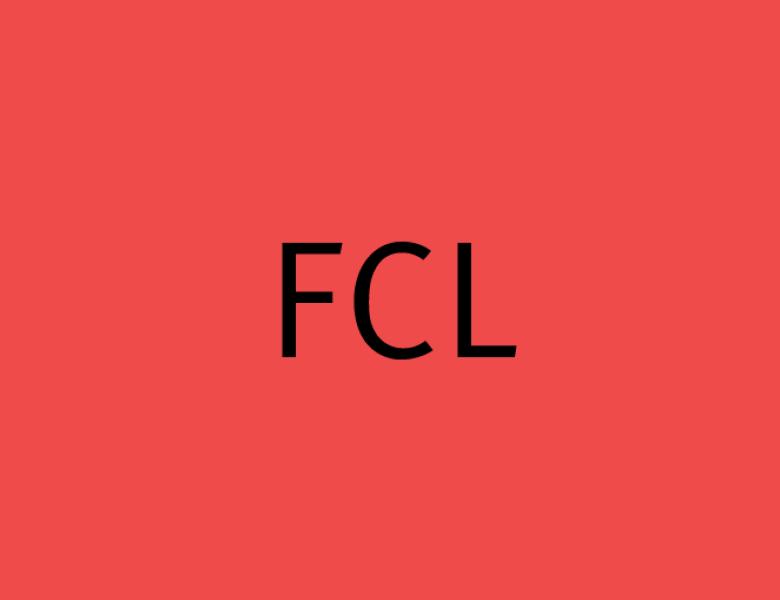
Collaborative learning systems often require collectively learning from multiple parties or agents in a decentralized manner. For such systems to be effective it is necessary to develop trustworthy learning schemes for entities participating in collaboration, particularly related to potential privacy and security risks. While decentralized systems offer a useful structure for decomposing machine learning workflows into modular components that can be augmented for end-to-end protection, decentralization alone does not ensure rigorous privacy or security guarantees, and may in fact introduce new threats to the participating entities. In addition, systems for federated and collaborative learning may present newfound challenges related to efficiency, accuracy, and usability for existing, theoretically-motivated approaches in privacy and security. There is thus a need to develop, extend, and analyze principled approaches for private and secure decentralized learning that can meet the needs of practical collaborative learning workloads. This workshop will focus on the theory and practice of private and secure algorithms for collaborative learning, drawing on researchers from privacy and security communities.