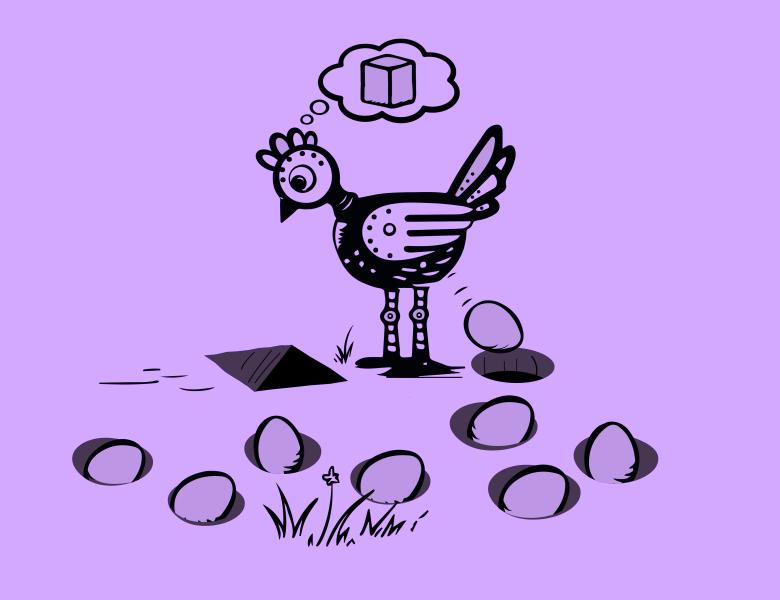
Abstract
Language model pretraining has been a remarkably strong recipe for cross-task and cross-domain generalization in NLP. However, these gains have come at the expense of control: we rarely control the training data for language models, and gaps between pretraining and our target evaluation lead to distribution shifts. We present two complementary approaches to control this gap — algorithmically filtering data to focus training on the most benchmark-relevant parts of the distribution, as well as adapting to new domains by synthesizing domain-specific pretraining data at scale.