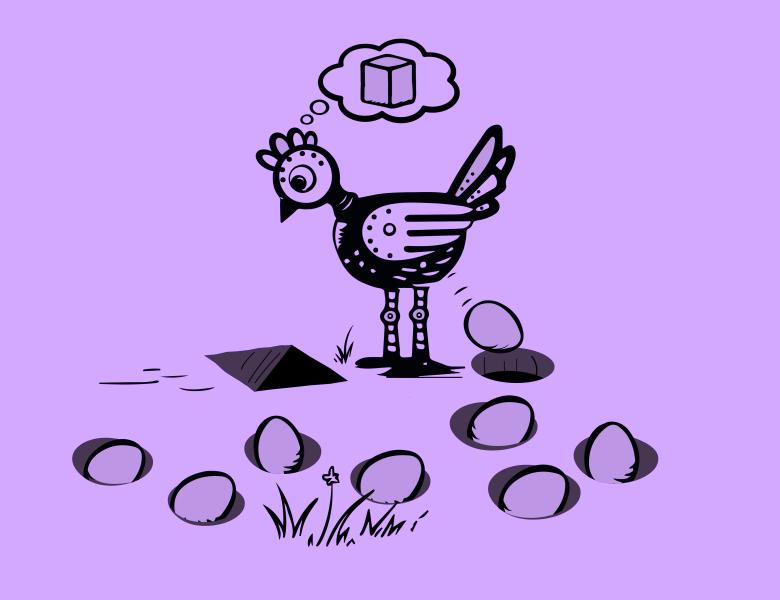
Abstract
We introduce recent theoretical development that elucidates the learning capabilities of Transformers, focusing on in-context learning as the main subject. First, regarding statistical efficiency and approximation ability, we show that Transformers can achieve the minimax optimality for in-context learning, and show superiority against non-pretrained methods. Next, in terms of optimization theory, we demonstrate that nonlinear feature learning for in-context learning can be done with optimization guarantee. More concretely, the objective becomes strict-saddle in a mean field setting, and if the target is a single index model, then its computational efficiency can be evaluated based on the information exponent of the true function.