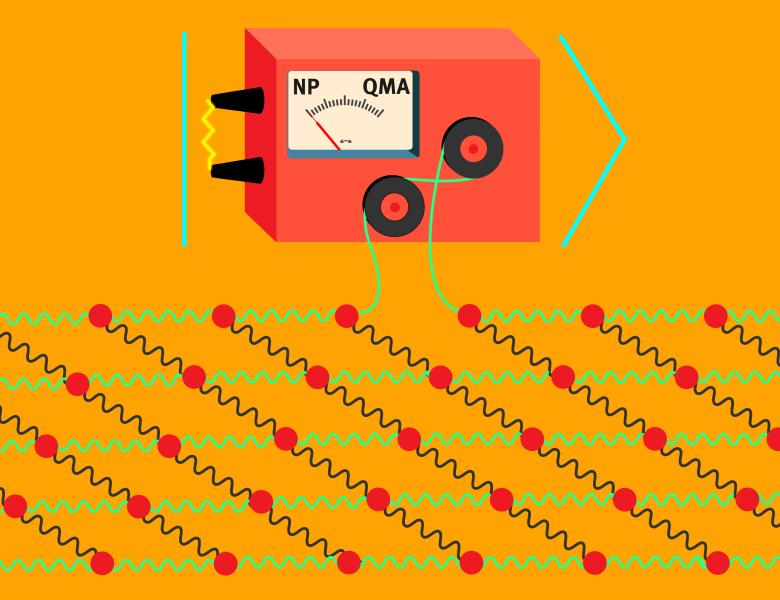
Abstract
Analog quantum machine learning leverages the natural dynamics of quantum systems to perform computations, making it uniquely suited for operation on current, noisy quantum hardware. In this context, we explore how a modern programmable quantum simulator embodies the necessary capabilities for executing diverse cognitive tasks, including multitasking, decision-making, and memory enhancement. A crucial advancement is the introduction of a foundational component for various learning architectures. I will illustrate the versatility of this approach by applying it to tasks such as calculating energy measurements and enhancements in quantum metrology and highlight how a hybrid quantum-classical approach can enhance the present-day applicability of quantum algorithms.