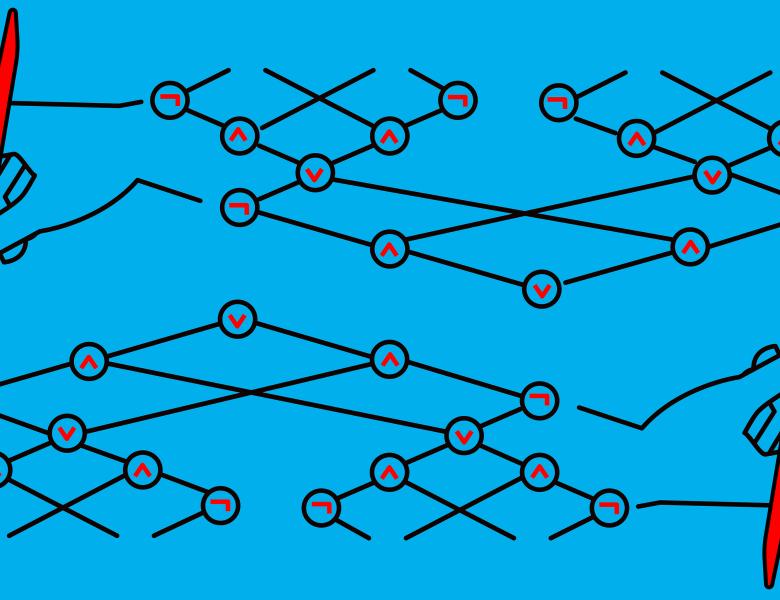
Abstract
We provide compelling evidence for the potential of hardness-vs.-randomness approaches to make progress on the long-standing problem of derandomizing space- bounded computation.
Our first contribution is a derandomization of bounded-space machines from hardness assumptions for classes of uniform deterministic algorithms, for which strong (but non-matching) lower bounds can be unconditionally proved. We prove one such result for showing that BPL = L “on average”, and another similar result for showing that BPSPACE[O(n)] = DSPACE[O(n)].
Next, we significantly improve the main results of prior works on hardness-vs.-randomness for logspace. As one of our results, we relax the assumptions needed for derandomization with minimal memory footprint (i.e., showing BPSPACE[S] ⊆ DSPACE[c · S] for a small constant c), by completely eliminating a cryptographic assumption that was needed in prior work.
A key contribution underlying all of our results is non-black-box use of the descriptions of space-bounded Turing machines, when proving hardness-to-randomness results. That is, the crucial point allowing us to prove our results is that we use properties that are specific to space-bounded machines.