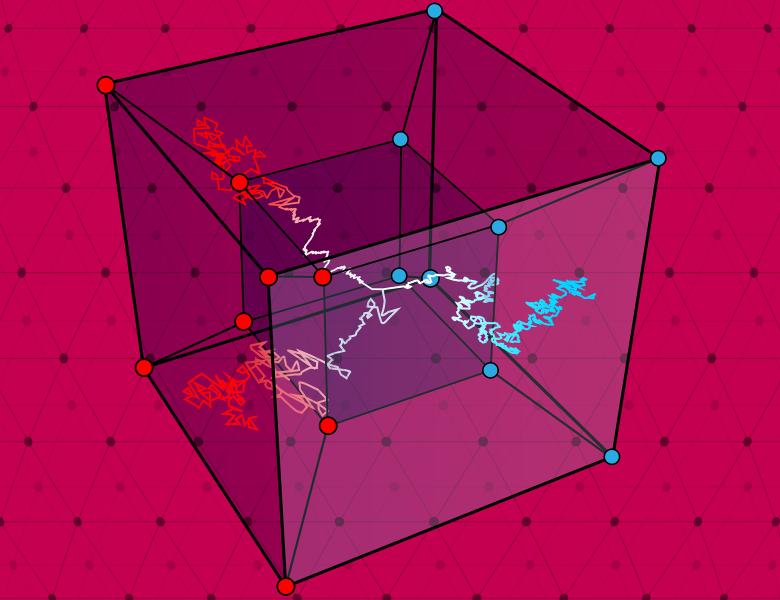
Abstract
We develop a framework for sampling from discrete distributions $\mu$ on the hypercube $\{\pm 1\}^n$ by sampling from continuous distributions supported on $\mathbb{R}^n$ obtained by convolution with spherical Gaussians. We show that for well-studied families of discrete distributions $\mu$, convolving $\mu$ with Gaussians yields well-conditioned log-concave distributions, as long as the variance of the Gaussian is above an $O(1)$ threshold. We then reduce the task of sampling from $\mu$ to sampling from tractable continuous distributions. Our reduction is based on a stochastic process widely studied under different names: the backward process in diffusion models, and stochastic localization. We discretize this process in a novel way that allows for high accuracy and parallelism. As our main application, we resolve open questions on the parallel sampling of distributions that admit parallel counting. We obtain the first polylogarithmic-time sampling algorithms for determinantal point processes, directed Eulerian tours, and more.
Joint work with Yizhi Huang, Tianyu Liu, Thuy-Duong Vuong, Brian Xu, Katherine Yu.