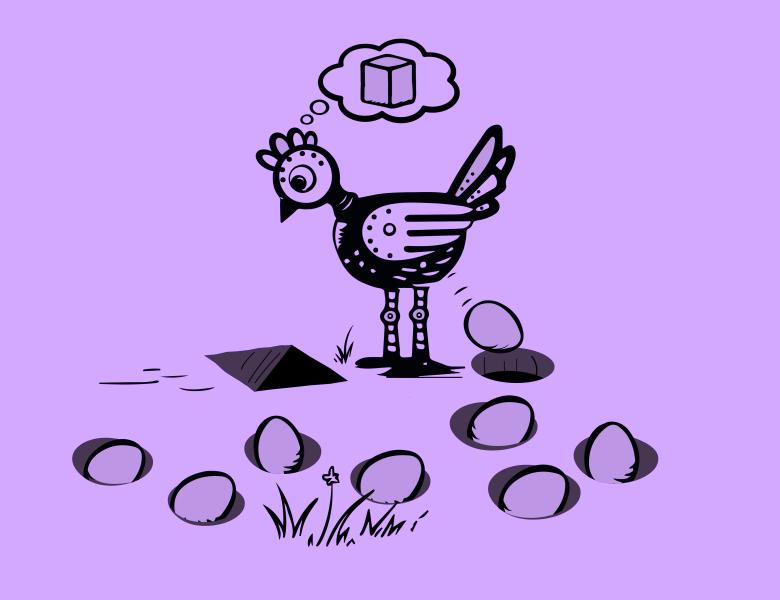
Abstract
Increasingly, practitioners are turning to machine learning (ML) to build predictive models that perform well under distribution shifts. However, empirical evidence shows that most predictive models lack robustness when facing these shifts at test time. In this talk, I will present my work on developing novel techniques to address these challenges.
I will focus on a major failure mode in robust ML: shortcut learning, where models learn unstable associations that fail as the test distribution shifts. I will present my research on causally-motivated regularization techniques designed to prevent shortcuts by encouraging models to conform to a specified causal structure. I will discuss scenarios where potential shortcuts are known beforehand and those where such knowledge is unavailable.