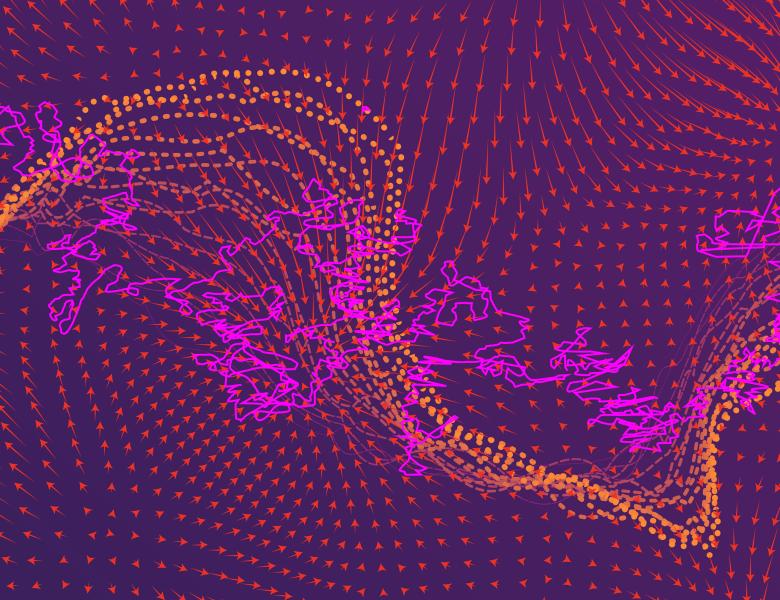
Abstract
Adversarial learning is a framework widely used by machine learning practitioners to enforce robustness of learning models. Despite the development of several computational strategies for adversarial learning and some theoretical development in the broader distributionally robust optimization literature, there are several theoretical questions about adversarial learning that remain relatively unexplored. One such question is to understand, in more precise mathematical terms, the type of regularization enforced by adversarial learning in modern settings like non-parametric classification as well as classification with deep neural networks. In this talk, I will present a series of connections between adversarial learning and several problems in the calculus of variations, geometric measure theory, and optimal control. All these connections aim at answering the question: what is the regularization effect induced by adversarial learning? In particular, for a family of non-parametric classification problems I will draw a connection between adversarial learning and perimeter minimization variational problems and mean curvature flow type evolution equations. Likewise, for a family of learning problems with deep neural networks, cast as control problems, I will discuss the form of the regularization effect of adversarial learning and some of its algorithmic implications. Throughout the talk I will highlight the fundamental intuition on adversarial learning that the powerful geometric interpretation of optimal transportation provides. This talk is based on joint works with Ryan Murray and Camilo A. García Trillos.