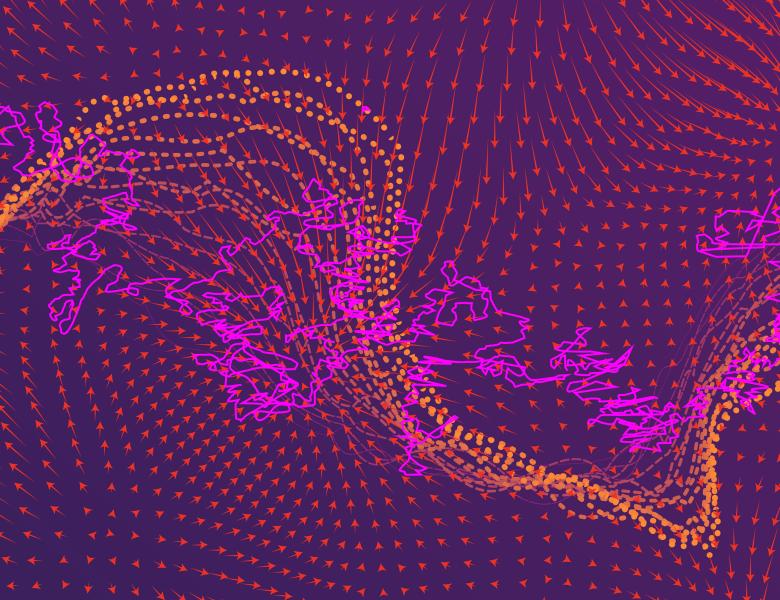
Abstract
Gradient-based sampling methods have been widely used in Bayesian inference. However, they suffer from computational inefficiency when applied to large-scale datasets, and the typical solution is to use mini-batch stochastic gradients to replace the full gradient. In this talk, I will present the convergence results of sampling from smooth and strongly log-concave for two families of gradient-based sampling methods: (1) underdamped Langevin Monte Carlo; and (2) Hamiltonian Monte Carlo, when combined with stochastic gradient estimators such as mini-batch stochastic gradients, control-variate stochastic gradients, and variance-reduced stochastic gradients. I will discuss the key proof ideas and techniques for these two families of algorithms, and highlight the similarities and differences between them. This talk is based on joint work with Difan Zou and Pan Xu.