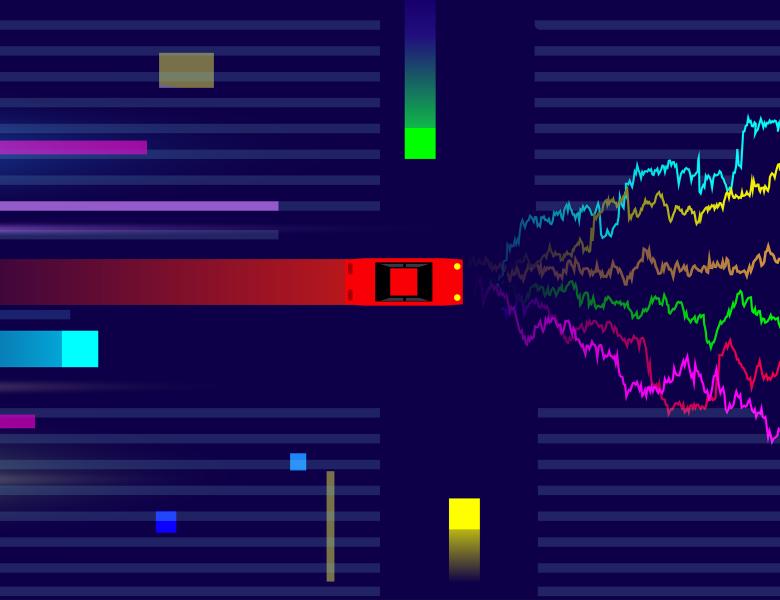
Abstract
We consider Pandora's box search problems with optional inspection and single-item or combinatorial selection. A decision maker is presented with a number of items, each of which contains an unknown price, and can pay an inspection cost to observe the item's price before selecting it. Under single-item selection, the decision maker must buy one item; under combinatorial selection, the decision maker must buy a set of items that satisfies certain constraints. In our optional inspection setting, the decision maker can buy items without first inspecting them. It is well known that search with optional inspection is harder than the well-studied mandatory inspection case, for which the optimal policy for single-item selection and approximation algorithms for combinatorial selection are known. We introduce a technique, called local hedging, for constructing policies with good approximation ratios in the optional inspection setting. Local hedging takes policies for the mandatory inspection setting and turns them into policies for the optional inspection setting. The cost of local hedging is an extra factor in the approximation ratio, which is instance-dependent but always at most 4/3. We thus obtain the first approximation algorithms for a variety of combinatorial selection problems in the optional-inspection setting, including matroid basis, matching, and facility location. Joint work with Laura Doval (Columbia Business School).