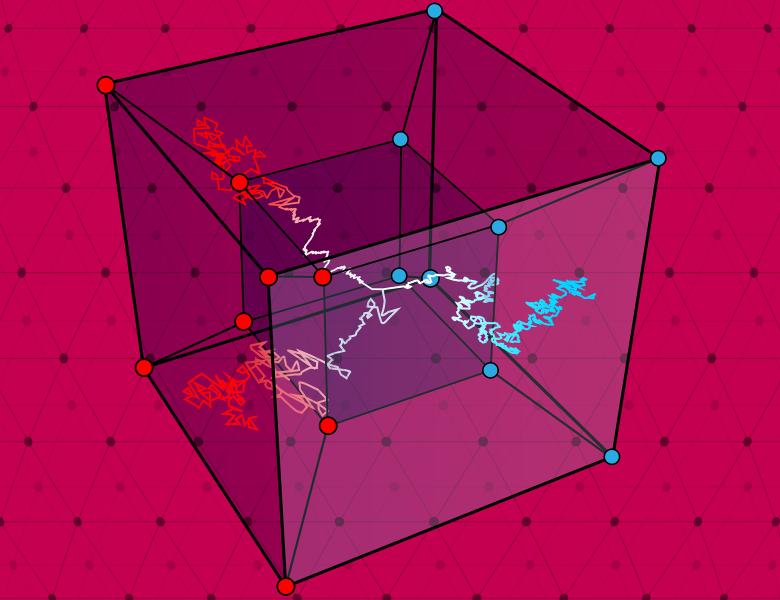
Abstract
We introduce localization schemes for analyzing the mixing time of Markov chains on continuous space and discrete hypercube. A localization scheme assigns every probability measure a martingale of probability measures which localize in space as time evolves. The use of localization schemes allows us to reduce the mixing time analysis on the original target distribution to that on many simpler transformed distributions.
After reviewing its connection to the existing literature on high dimensional concentration and convex geometry, we first explain Eldan's stochastic localization on Euclidean space. Next, we demonstrate how stochastic localization can be used for sampling Ising models in the uniqueness regime on the discrete hypercube, and discuss the differences between working on discrete and continuous spaces. This observation regarding the differences motivates us to introduce new Poisson-process-driven negative fields localization schemes for analyzing Glauber dynamics for sampling the hardcore model.