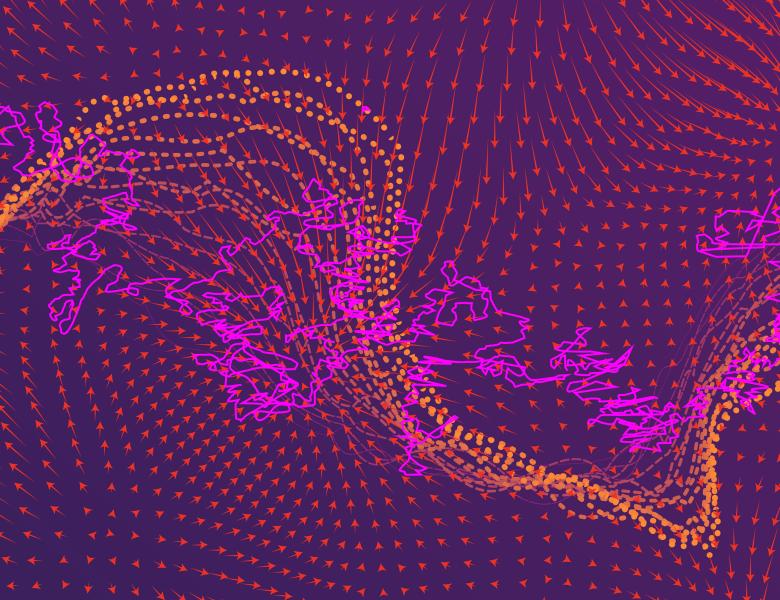
Abstract
Wasserstein GANs (WGANs) are based on the idea of minimising the Wasserstein distance between a real and a generated distribution. We provide an in-depth mathematical analysis of differences between the theoretical setup and the reality of training WGANs. In this work, we gather both theoretical and empirical evidence that the WGAN loss is not a meaningful approximation of the Wasserstein distance. In addition, we argue that the Wasserstein distance is not a desirable loss function for deep generative models. We conclude that the success of WGANs can be attributed to the failure to approximate the Wasserstein distance. (https://arxiv.org/pdf/2103.01678.pdf)