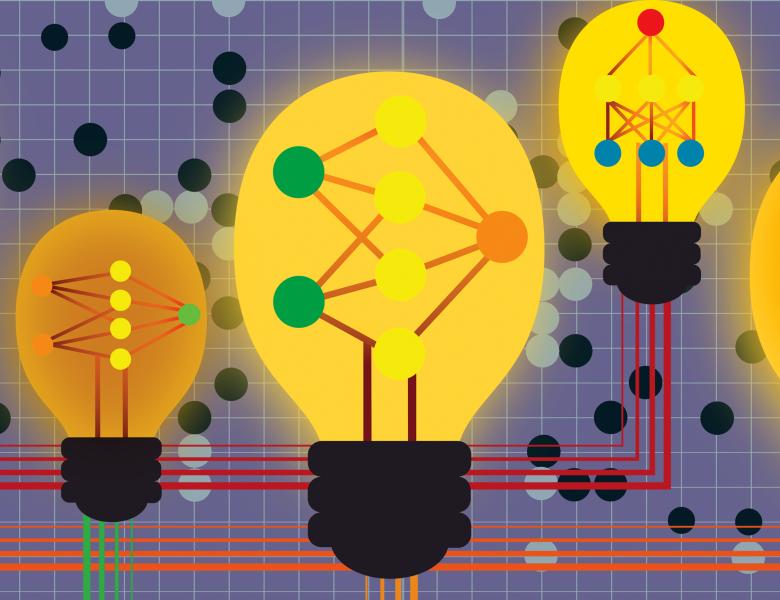
Abstract
The conditional moment problem is a powerful formulation for describing structural/causal parameters in terms of observables, a prominent example being instrumental variable (IV) regression. Standard approaches rely on reducing this to a finite (possibly growing) set of marginal moments and applying an optimally-weighted generalized method of moments (OWGMM), or other sieve-based methods. Many recent works have proposed a variety of minimax estimators that instead consider infinitely-many moments. These permit the use of flexible function classes such as neural nets, but they lack the celebrated efficiency of OWGMM and do not permit inference. Motivated by a variational reformulation of OWGMM that accounts for the optimal weighting, we define a general class of minimax estimators for the conditional moment problem that we term the variational method of moments (VMM). Crucially, we establish that certain instantiations of VMM with infinite function classes are in fact asymptotically normal and also semiparametrically efficient in the full conditional moment model. And, we provide asymptotically-valid inference algorithms based on the same kind of variational reformulations. We demonstrate our proposed estimation and inference algorithms for IV and policy learning.