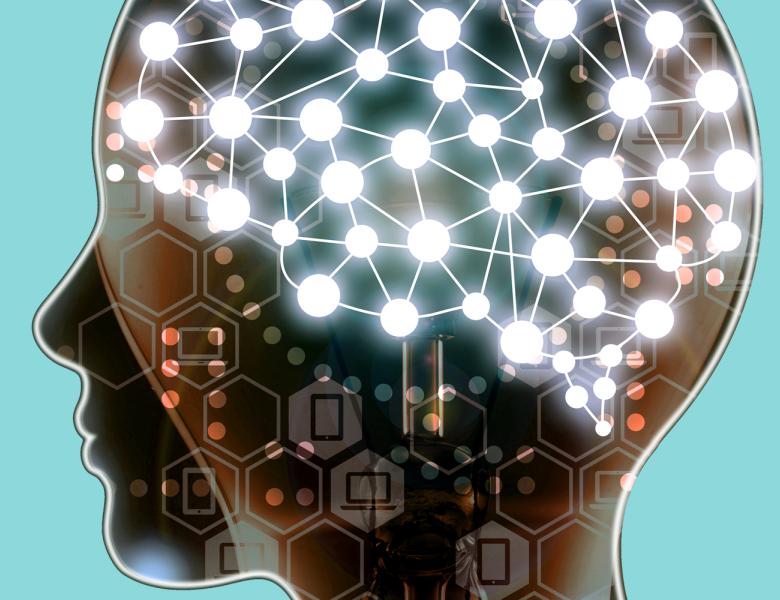
Abstract
It is well known that, at a random initialization, as their width approaches infinity, neural networks can be well approximated by Gaussian processes. We quantify this phenomenon by providing non-asymptotic convergence rates in the space of continuous functions. In the process, we study the Central Limit Theorem in high and infinite dimensions, as well as anti-concentration properties of polynomials with random variables