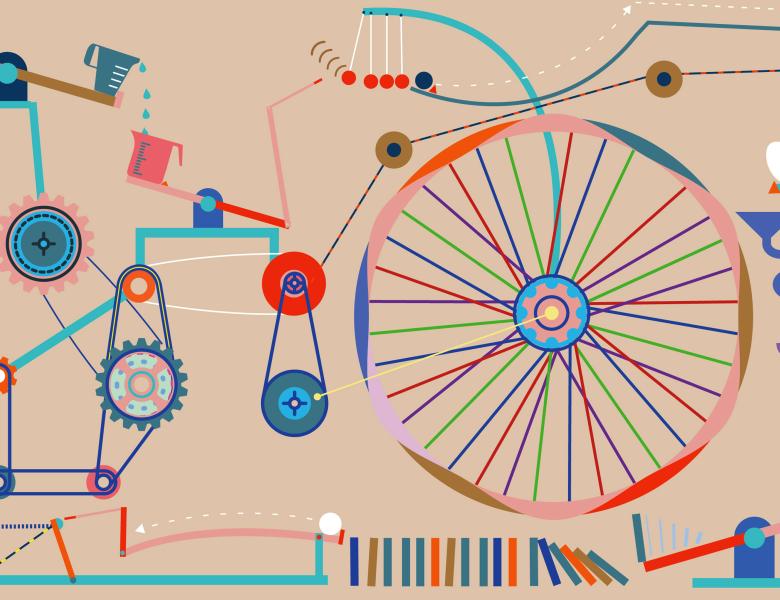
Abstract
It has been understood for more than 30 years that DAG models with hidden variables give rise to non-parametric (“Verma”) constraints that generalize conditional independence. The nested Markov model is a class of graphical models associated with acyclic graphs containing directed and bidirected edges that encode all of the non-parametric equality constraints implied by DAGs with latent variables. In this talk I will first define the nested Markov model and the associated global property. I will then describe simple rules for reasoning with such constraints. Finally I will define a local property, explaining why this construction is harder than for ordinary independence models.