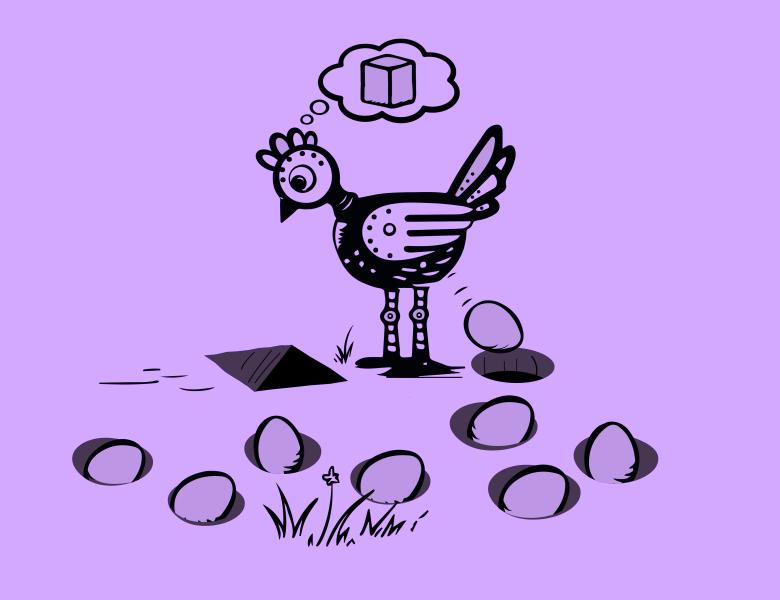
Abstract
The rise of models pre-trained with self-supervision has transformed AI and ML in the last few years. However, their working largely remains a mystery; classical learning theory cannot explain why pre-training on an unsupervised task can help many different downstream tasks. This talk will first investigate the role of pre-training losses in extracting meaningful structural information from unlabeled data, especially in the infinite data regime. Concretely, I will show that the contrastive loss can give rise to embeddings whose Euclidean distance captures the manifold distance between raw data (or, more generally, the graph distance of a so-called positive-pair graph). Moreover, directions in the embedding space correspond to relationships between clusters in the positive-pair graph. Then, I will touch on other elements that seem necessary for a sharp explanation of the behavior of practical pre-trained models: inductive bias of architectures and implicit bias of optimizers. I will introduce two recent papers that (1) strengthen the previous theoretical framework by incorporating the inductive bias of architectures and (2) demonstrate the implicit bias of optimizers in pre-training, even with infinite pre-training data, empirically and theoretically.