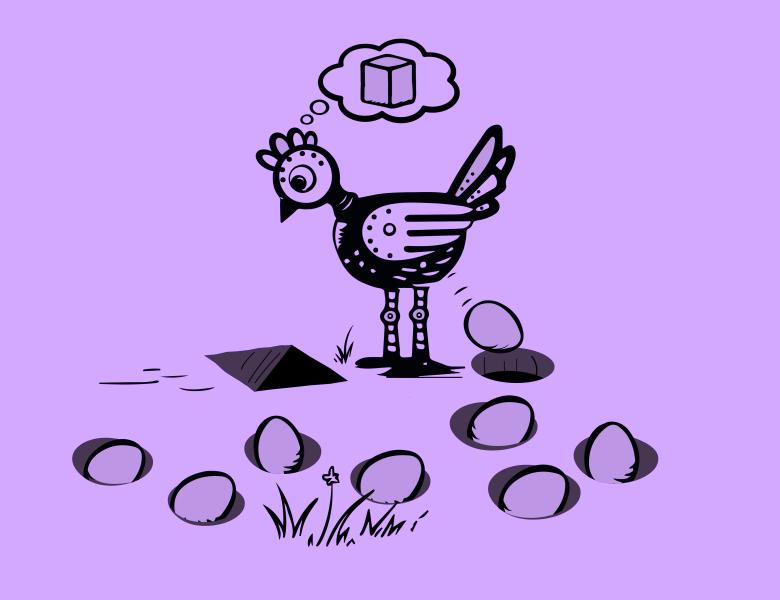
Abstract
In this talk, we will focus on the problem of sequential prediction in the stochastic setting subject to adversarial interference (or distribution shift), where clean-label adversarial (or out-of-distribution) examples can be injected at any point. Traditional algorithms, designed for purely stochastic data, fail to generalize effectively in the presence of such injections, often leading to erroneous predictions. Conversely, approaches that assume fully adversarial data yield overly pessimistic bounds, offering limited practical utility. To overcome these limitations, we will introduce a new framework that allows the learner to abstain from making predictions at no cost on adversarial injections, thereby asking the learner to make predictions only when it is certain. We will design algorithms in this new model that retain the guarantees of the purely stochastic setting even in the presence of arbitrarily many adversarial examples. We will conclude with several exciting open questions that our new framework posits.