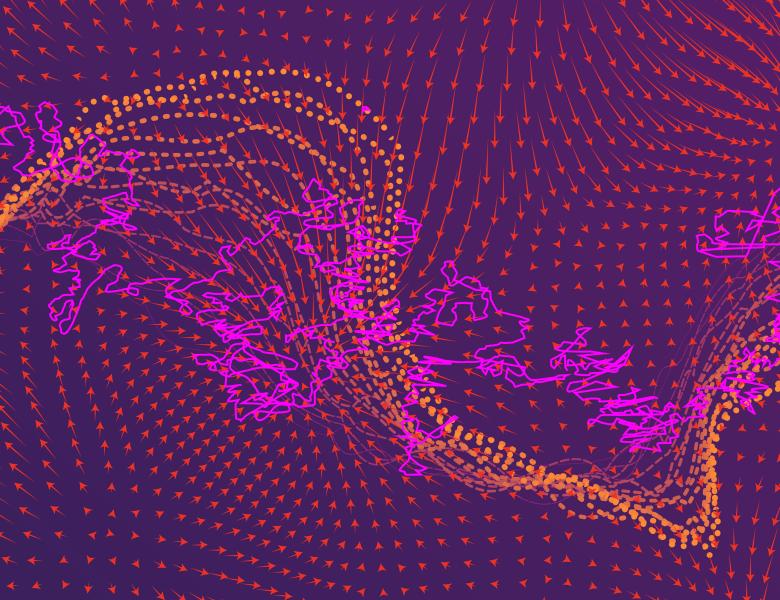
Abstract
We give algorithms for sampling several structured logconcave families to high accuracy. We further develop a reduction framework, inspired by proximal point methods in convex optimization, which bootstraps samplers for regularized densities to generically improve dependences on problem condition number from polynomial to linear. A key ingredient in our framework is the notion of a ``restricted Gaussian oracle'' (RGO) for function g, which is a sampler for distributions whose negative log-likelihood sums a quadratic (in a multiple of the identity) and g. By combining our reduction framework with our new samplers, we obtain the state-of-the-art bounds for sampling composite densities and finite-sum densities to high accuracy in total variation distance.