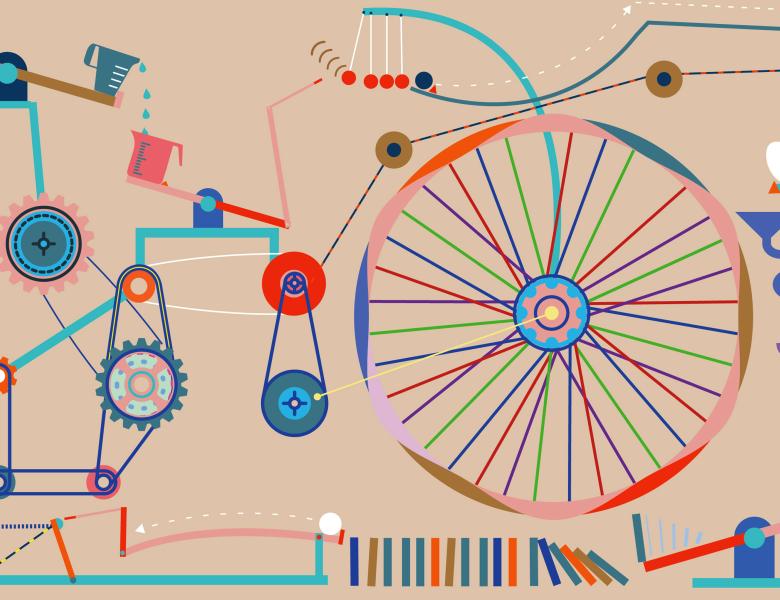
Abstract
Causal contributions measure the strengths of different causes to a target quantity. Understanding causal contributions is important in empirical sciences and data-driven disciplines since it allows to answer practical queries like “what are the contributions of each cause to the effect?” In this talk, we will look at a principled method for quantifying causal contributions that starts from the desiderata of properties (axioms) that causal contribution measures should satisfy and constructs a unique method (do-Shapley) satisfying these properties. Next, we develop a criterion under which the do-Shapley values can be efficiently inferred from non-experimental data. Finally, we will discuss various do-Shapley estimators (DML, IPW-based etc.) with focus on consistency, computational feasibility, and statistical robustness. Joint work with Yonghan Jung, Jin Tian, Dominik Janzing, Patrick Bloebaum, and Elias Bareinboim