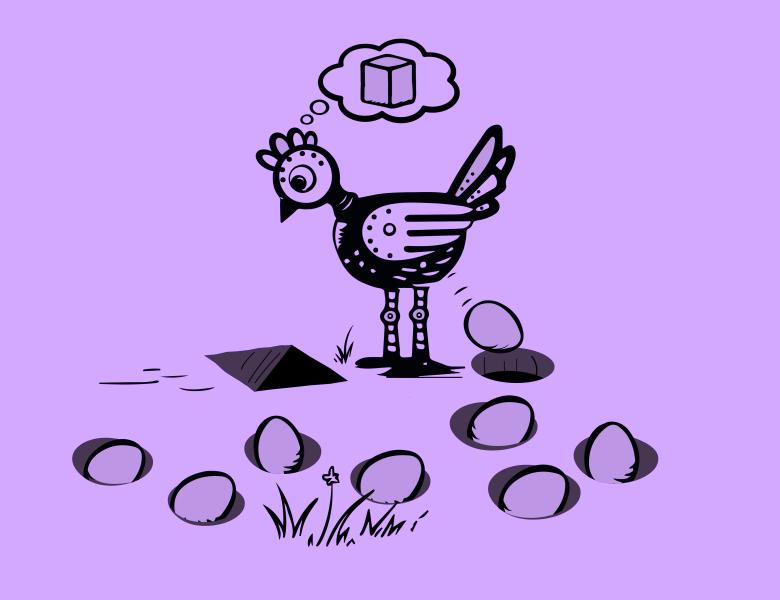
Abstract
Foundation models like GPT-4 have dramatically altered the modern work landscape for many industries reliant on language tasks, but no equivalent model exists yet for scientific applications. Incorporating foundation models into research workflows could enable unprecedented discoveries that connect traditionally distinct scientific sub-disciplines. However, mainstream foundation models trained on human-scale datasets will be insufficient for analyzing most scientific phenomena -- a foundation model for science will require special consideration for the requirements of scientific datasets, especially those with wide dynamic ranges.
In this talk , I will introduce the Polymathic AI initiative: our goal is to accelerate the development of versatile foundation models tailored for numerical datasets and scientific machine learning tasks. The challenge we are undertaking is to build AI models which leverage information from heterogeneous datasets and across different scientific fields, which, contrary to domains like natural language processing, do not share a unifying representation (i.e., text). Such models can then be used as strong baselines or be further fine-tuned by scientists for specific applications. This approach has the potential to democratize AI in science by providing off-the-shelf models that have stronger priors (i.e., background knowledge) for shared general concepts such as causality, measurement, signal processing, and even more specialized shared concepts like wave-like behavior, which otherwise would need to be learned from scratch.
I will present our initial papers and projects, including large scientific datasets designed for large scale training "MultiModal Universe" and "The Well".