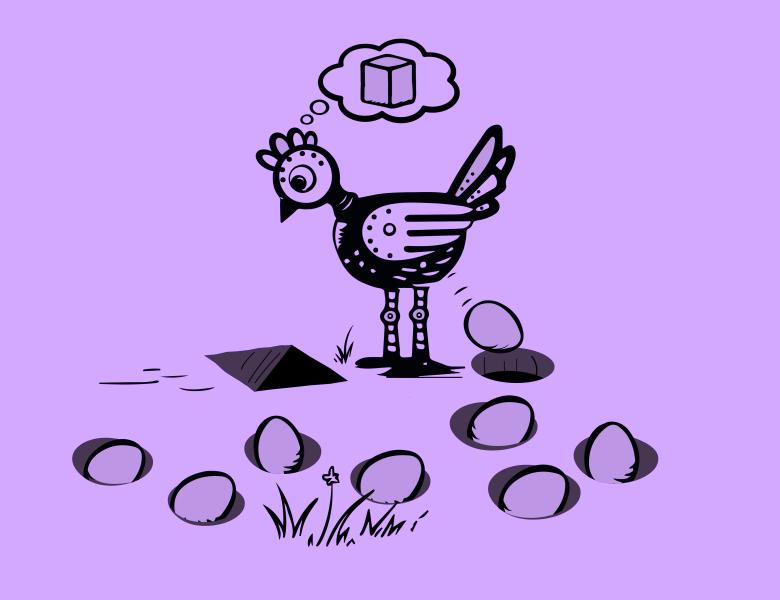
Abstract
A possible high-level description of statistical learning is that it aims to learn about some unknown probability distribution (``environment”) from samples it generates (``training data”). In this talk I will discuss two major research directions concerning guaranteed generalizations from finite samples. First, I will address learning under a common prior knowledge assumption - settling the question of the sample complexity of learning mixtures of Gaussians. Secondly, I will address the challenge of characterizing which families of distributions are learnable (with PAC style generalization guarantees). I will show that, in contrast with binary classification prediction and many other common ML tasks, learnability of distribution families cannot be characterized by a combinatorial dimension. The first part is based on joint work with Hasan Ashiani, Nick Harvey, Chris Law, Abas Merhabian and Yaniv Plan and the second part on work with my student Tosca lechner.