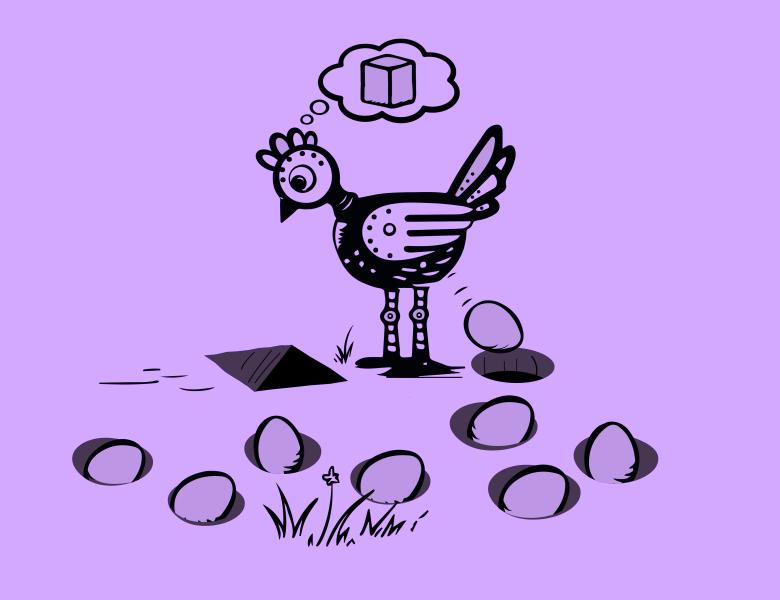
Abstract
Classical statistical decision theory treats the relationship between prediction error, generalization gap (which the statistics literature calls optimism), and model complexity from a point of view that treats the covariates X as fixed, nonrandom values. This fixed-X perspective delivers a number of insights, many of which are well-known to statisticians, but perhaps less well-known to researchers in machine learning. This talk reviews the classical statistics literature, and then emphasizes the ways in which this fixed-X theory is insufficient to the explain prediction error, generalization gap, and model complexity in a random-X setting---which is the predominant view in machine learning---especially as a predictive model becomes flexible enough to interpolate the training data. Finally, we show how to reinterpret some of the fixed-X classical statistics concepts in order to extend them to a random-X setting.