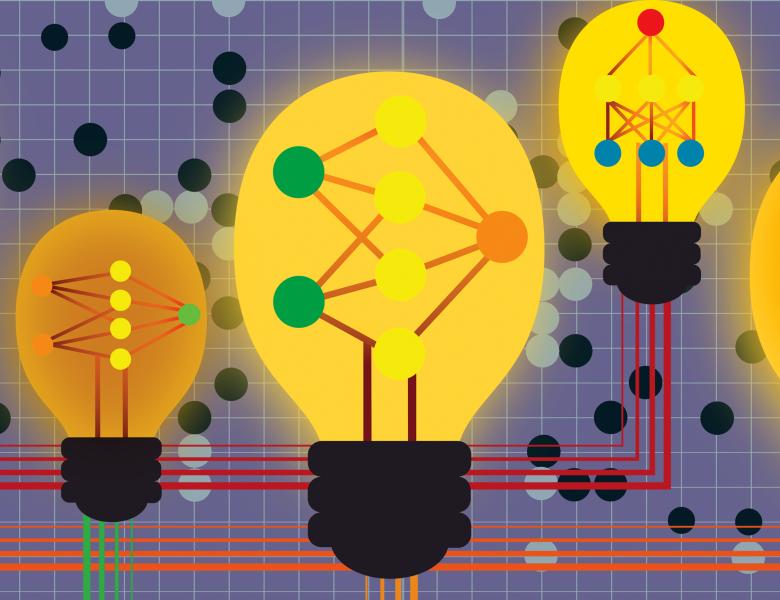
Abstract
Today I will be discussing some of the challenges and lessons learned in partner modeling in decentralized multi-agent coordination. We will start with discussing the role of representation learning in learning effective conventions and latent partner strategies and how one can leverage the learned conventions within a reinforcement learning loop for achieving coordination, collaboration, and influencing. We will then extend the notion of influencing beyond optimizing for long-horizon objectives, and analyze how strategies that stabilize latent partner representations can be effective in reducing non-stationarity and achieving a more desirable learning outcome. Finally, we will formalize the problem of decentralized multi-agent coordination as a collaborative multi-armed bandit with partial observability, and demonstrate that partner modeling strategies are effective approaches for achieving logarithmic regret.