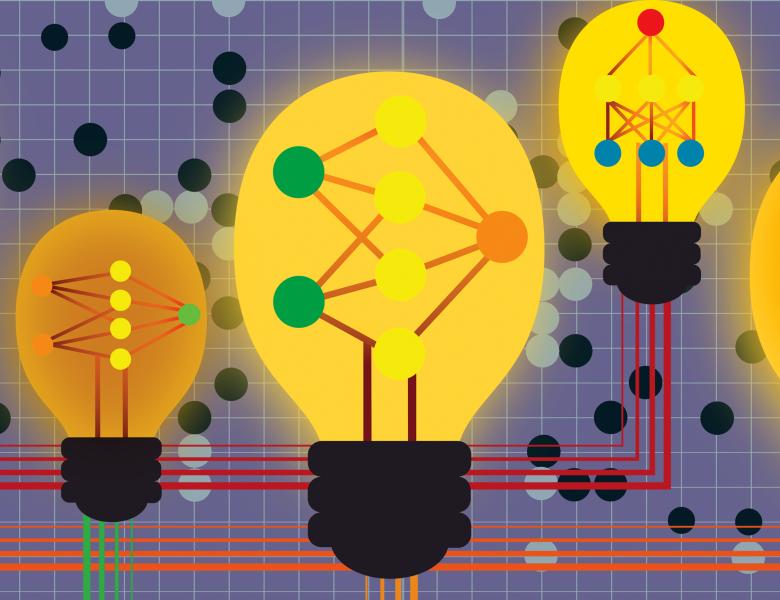
Abstract
We'll start by describing a new paradigm in reinforcement learning called nonstochastic control, how it relates to existing frameworks, and survey efficient gradient-based methods for regret minimization in this model. We then proceed to describe recent work on multi-agent learning based on regret minimization methods that reach an equilibrium. We'll conclude with remaining challenges and potential directions for further research.