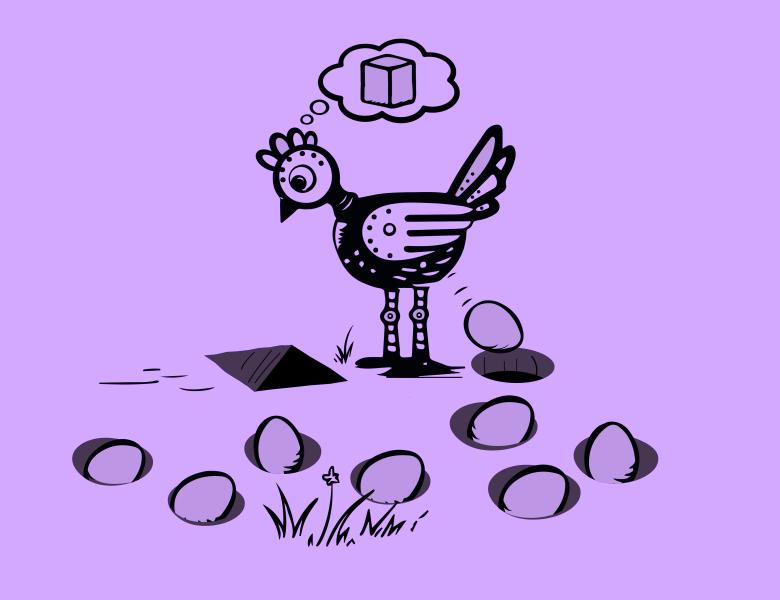
Abstract
While many fundamental problems in statistical learning have been studied by both statisticians and theoretical computer scientists alike, one sometimes observes a bifurcation of perspectives: statisticians naturally adopt an "average-case" approach — e.g., assuming i.i.d. draws from an underlying distribution — whereas computer scientists emphasize the limits of learning in worst-case scenarios. This talk will explore several such examples, outlining similarities and differences between the approaches and, in particular, highlighting the benefits of adopting an average-case perspective for analyzing problems that were previously shown to be intractable in the worst case.
For the full event description, please visit https://simons.berkeley.edu/events/worst-average-case-statistical-probl…