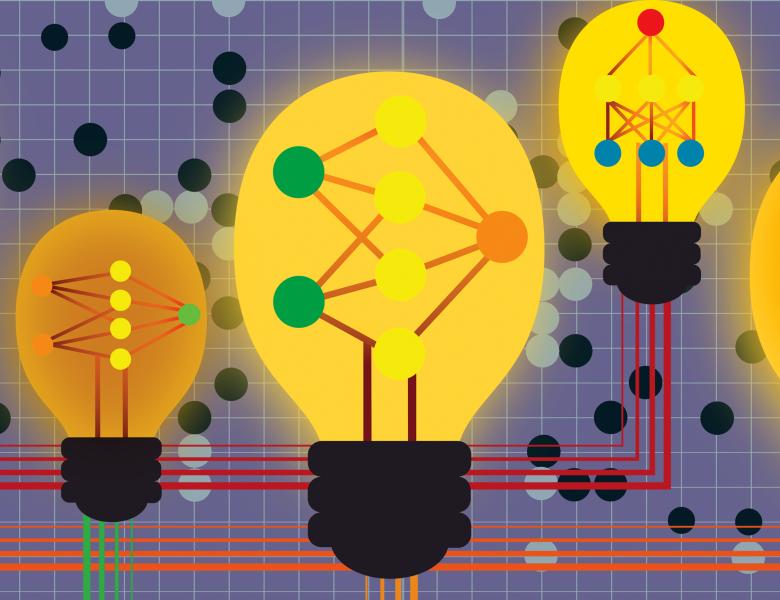
Abstract
In this paper we solve the problem of no-regret learning in general games. Specifically, we provide a novel, simple and efficiently computable learning algorithm, Clairvoyant Multiplicative Weights Updates (CMWU), that achieves constant regret in all normal-form games with fixed step-sizes. The cumulative regret of our algorithm provably decreases linearly as the step-size increases. Our findings depart from the prevailing paradigm that vanishing step-sizes are a prerequisite for low regret as championed by all state-of-the-art methods to date. Our arguments extend well beyond normal-form games with little effort.