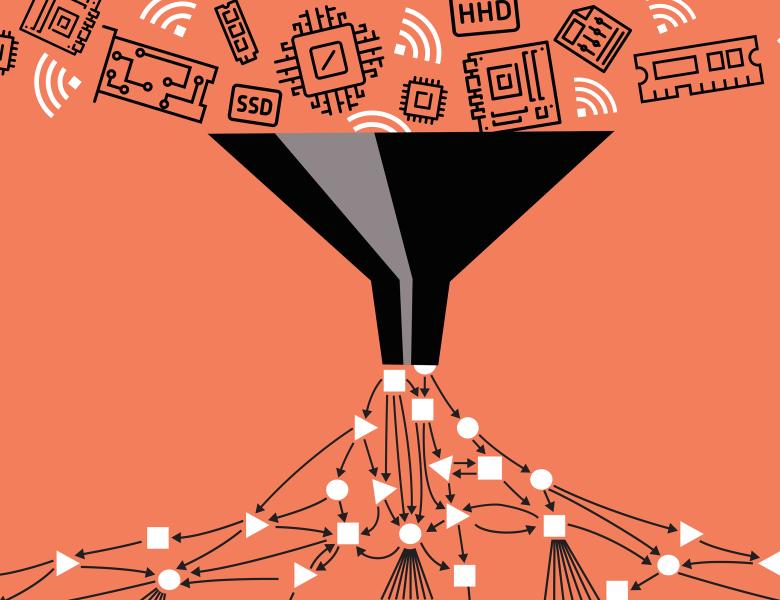
Abstract
In supervised machine learning, in an abstract sense, a concept in a given reference class has to be inferred from a small set of labeled examples. Machine teaching refers to the inverse problem, namely the problem to compress any concept in the reference class to a "teaching set" of labeled examples in a way that the concept can be reconstructed. The goal is to minimize the worst-case teaching set size taken over all concepts in the reference class, while at the same time adhering to certain conditions that disallow unfair collusion between the teacher and the learner.
In this presentation, two intuitive notions of collusion-freeness are discussed and mapped to teaching models that are optimal with respect to these notions of collusion-freeness. In particular, these two models will be compared regarding their complexity of teaching, which is formulated in terms of the worst-case number of examples required for teaching any concept in a given concept class.