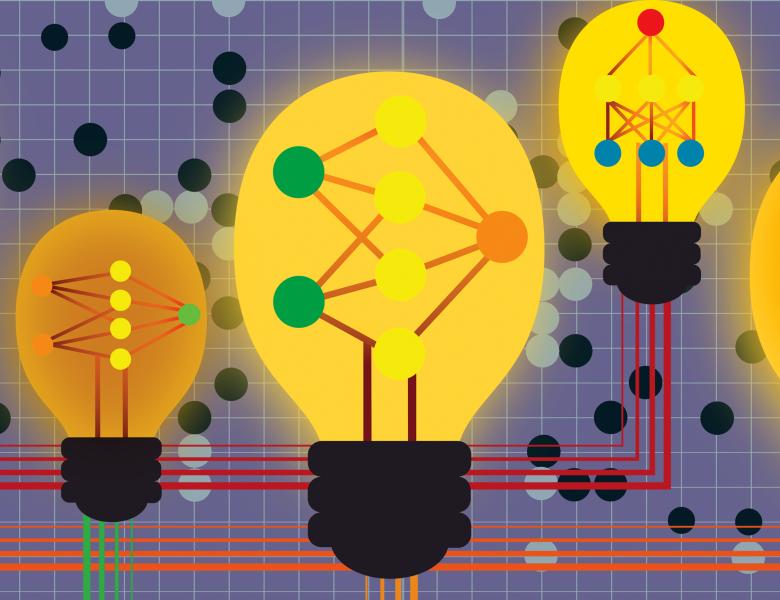
Abstract
Learning from repeated play in a fixed two-player zero-sum game is a classic problem in game theory and online learning. This talk focuses on a natural yet underexplored variant of this problem where the game payoff matrix changes over time, possibly in an adversarial manner. In the first part of the talk, I will discuss what the appropriate performance measures are for this problem (and argue that some measures from prior works might be unreasonable). In the second part of the talk, I will present a new parameter-free algorithm that simultaneously enjoys favorable guarantees under three different performance measures. These guarantees are adaptive to different non-stationarity measures of the payoff matrices and, importantly, recover the best known results when the payoff matrix is fixed. I will conclude the talk by discussing some future directions on the extensions to multi-player bandits and reinforcement learning.