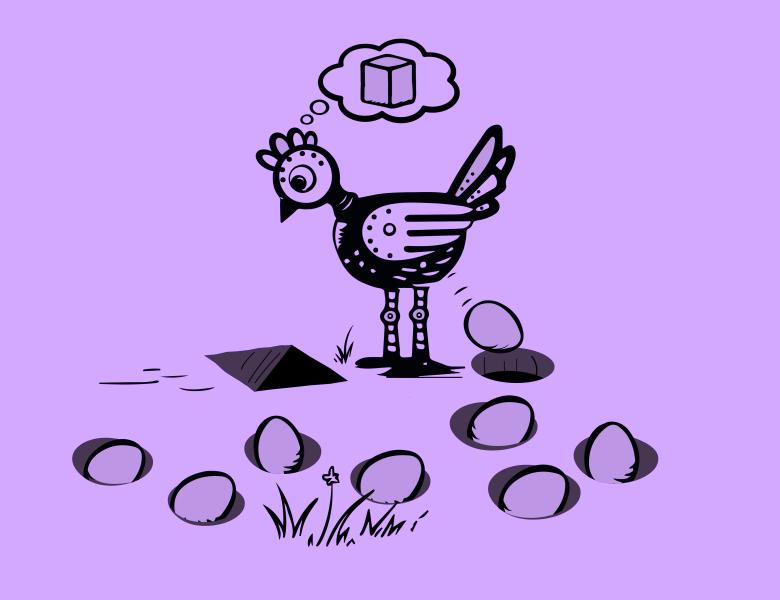
Abstract
In this talk, I will introduce the framework of multi-objective learning. This framework that acts as a unifying paradigm for addressing needs such as robustness, collaboration, and fairness aims to optimize a set of complex and unstructured objectives from only a small amount of sampled data. I will also discuss how the multi-objective learning paradigm relates to the classical and modern considerations in machine learning broadly, such as generalization, introduce technical tools with versatile provable guarantees, and empirical evidence for its performance on a range of important benchmarks.