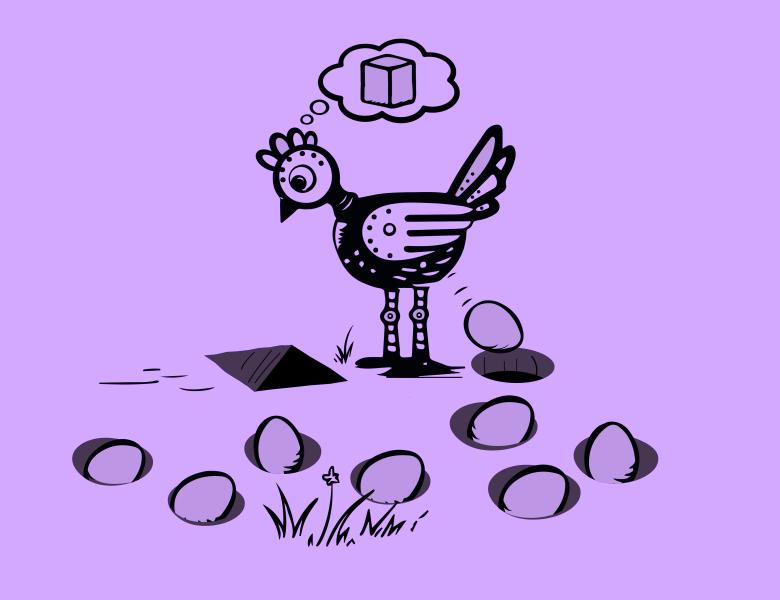
Abstract
Compositionality is considered a central property of human language. One key benefit of compositionality is the generalization it enables---the production and comprehension of novel expressions analyzed as new compositions of familiar parts. Whether artificial neural networks (ANNs) can generalize in such a way, and if so, the conditions under which such a generalization is achieved have been a longstanding line of inquiry in Cognitive Science. In this talk, I will discuss several semantic parsing tests we proposed to evaluate compositional linguistic generalization in ANNs, reviewing modeling results from the past few years and comparing them to human generalization patterns. In short, models can match human patterns in cases where only lexical substitution of known examples is required, but fail to do so when the generalization targets are structurally novel without being augmented with targeted structural scaffolding. In addition to this general picture, I will further highlight the difficulty of testing generalization in the current modeling landscape without open access to the training data, as well as the need and opportunity for better understanding structural generalization in humans.