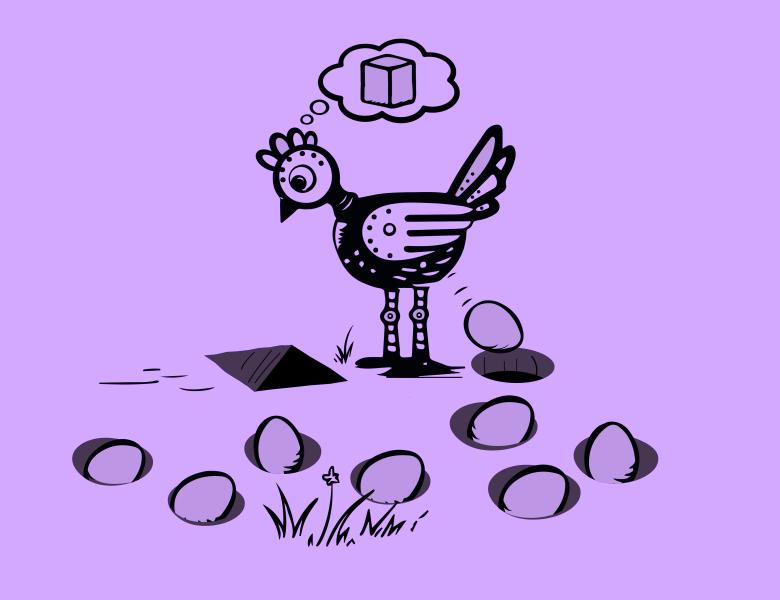
Abstract
Generalization is the central topic of machine learning and data science. What patterns can be learned from observations and how can we be sure that they extend to future, not yet seen, data? I will try to outline the arc of recent developments in understanding of generalization in machine learning. These changes occurred largely due to empirical findings in neural networks which necessitated revisiting theoretical foundations of generalizations. Classically, many analyses relied on the assumption that the training loss was an accurate proxy of the test loss. This turned out to be unfounded as good practical predictors frequently have training loss that is much lower than the test loss. Theoretical developments, such as analyses if interpolation and double descent have recently shed light on that issue. In view of that, a common practical prescription has become to mostly ignore the training loss and to adopt early stopping -- to stop the model training once the validation loss plateaus. Recent discovery of emergent phenomena like grokking show that this practice is also not generally justifiable as at least in some settings, the test loss up to a certain iteration may not be predictive of the test loss itself just a few iterations later. I will discuss why this presents a fundamental challenge to both theory and practice of machine learning, and attempt to describe the current state of affairs.