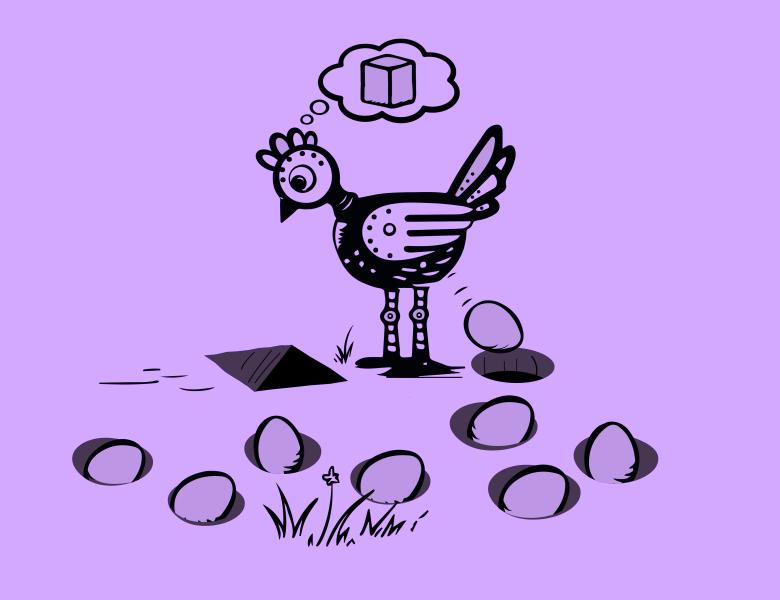
Abstract
This talk presents a framework for adaptation grounded in the concept of discrepancy, extending to both unsupervised and weakly supervised settings. We provide a theoretical analysis of sample reweighting techniques, with bounds that guide the design of effective algorithms for domain adaptation, especially when labeled target data is limited or absent. Experimental results will illustrate the efficacy of these algorithms, with comparisons to several baselines that highlight the robustness of our approach.