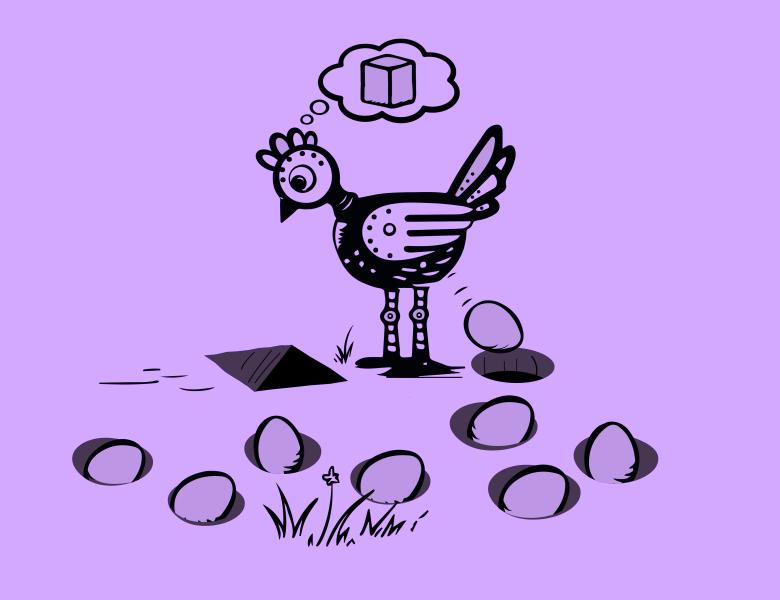
Abstract
Humans can answer questions such as “Did Aristotle own a coffee making machine?” without having seen many or even any examples that showed Aristotle owning or missing a kitchen appliance. We suggest that humans answer such questions by chaining together beliefs that are each learned from examples in the PAC sense, and then chained in a way that is provably sound. Following the robust logic framework, soundness is interpreted here in the probabilistic sense that if the beliefs that are combined are each supported by the training data to a certain probability, such as 90%, then the conclusion of the chaining will be provably also supported by the training data to some other level, such as 80%. Just as PAC learning is principled, but for its success needs something from the world, namely that the concept is learnable from the available data, sound chaining as just described is also principled, but needs from the world that the component beliefs are learnable separately from the available data to sufficient accuracy. If the world is modular in that it abounds with rules that are separately learnable from data over different limited feature sets, then the chaining process will make predictions that are technically out-of-distribution but still principled. We shall discuss the power of the robust logic framework in this context, and its relevance to large language models.