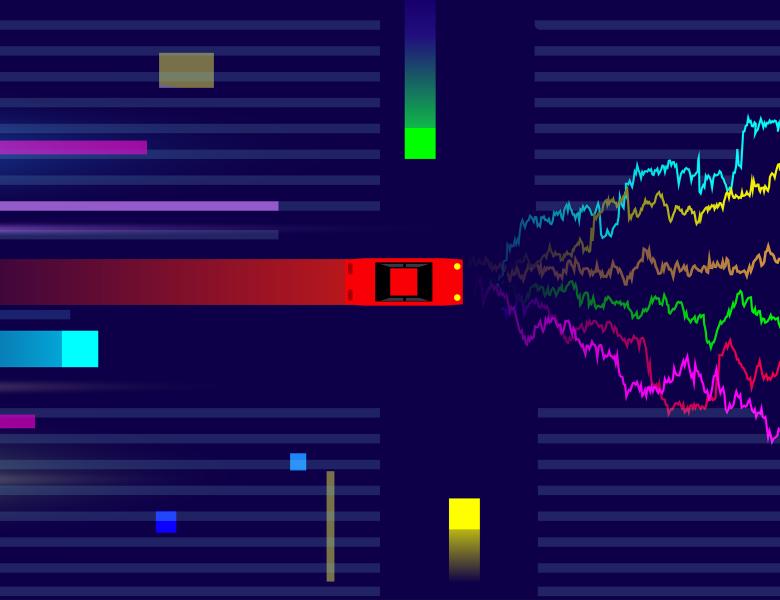
Abstract
This talk studies zero-sum matrix games where both players observe stochastic outcomes of their respective plays, where the underlying process defining the outcomes is initially unknown. Through repeated play, the players infer the underlying process and attempt to improve their strategies. We consider two distinct cases: 1) where the learner controls both players and the objective is to identify an approximate Nash equilibrium of the game as fast as possible, and 2) where the learner controls just the first player and the objective is to obtain as much reward as possible at the second player's expense. In both cases, we provide instance-dependent guarantees. For the second case, we define conditions under which logarithmic regret is attainable even against an adaptive adversary.