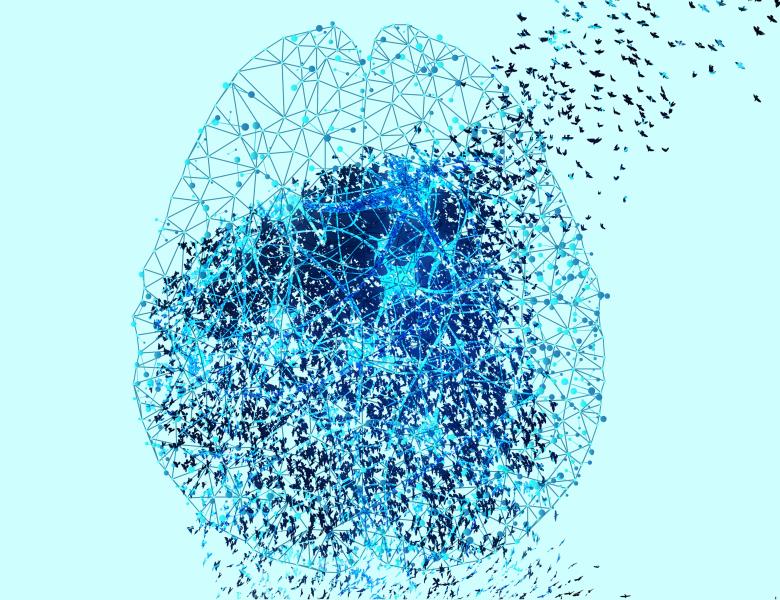
Abstract
The world is structured in countless ways. When cognitive and machine models respect these structures, by factorizing their modules and parameters, they can achieve remarkable efficiency and generalization. In this talk, I will discuss my work investigating the factorizations of objects, relations, and physics to support flexible physical problem-solving in both minds and machines. My research suggests that these ingredients can explain complex cognitive phenomena such as how people effortlessly learn to use new tools, and complex behaviours in machines such as highly realistic simulation and tool innovation. By taking better advantage of problem structure, and combining it with general-purpose methods for statistical learning, we can develop more robust and data-efficient machine agents while also better explaining how natural intelligence learns so much from so little.