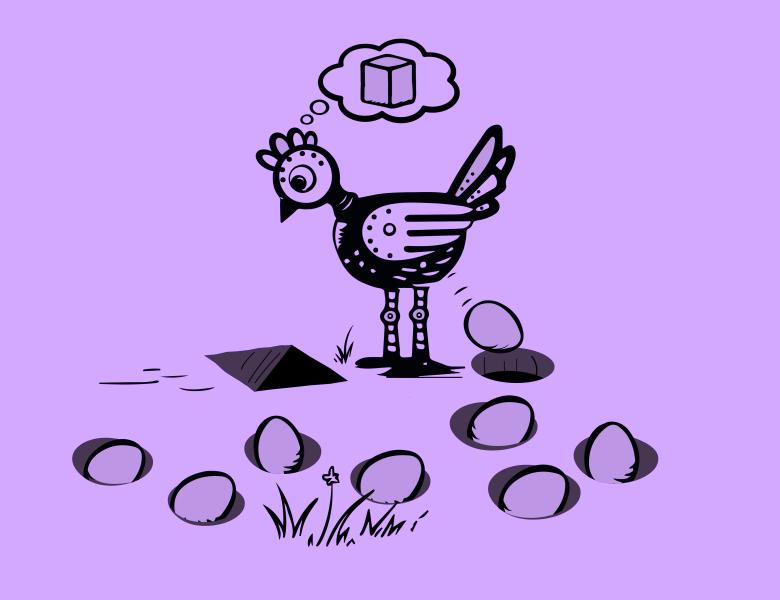
Abstract
When we try to mathematically derive optimal generalization bounds for large-capacity models, we naturally come to generalization bounds with the apparently unintuitive aspect that they feature different test and training losses. Relatedly, learned models may implicitly seek to optimize an objective which is different from the apparent training loss they are given. In some cases, the test loss which emerges is non-obvious and has unusual features like being discontinuous. We discuss these phenomena, the connection to the literature on Moreau envelopes, high dimensional asymptotics, and benign overfitting, and the special role of sqrt-Lipschitz losses in this theory.