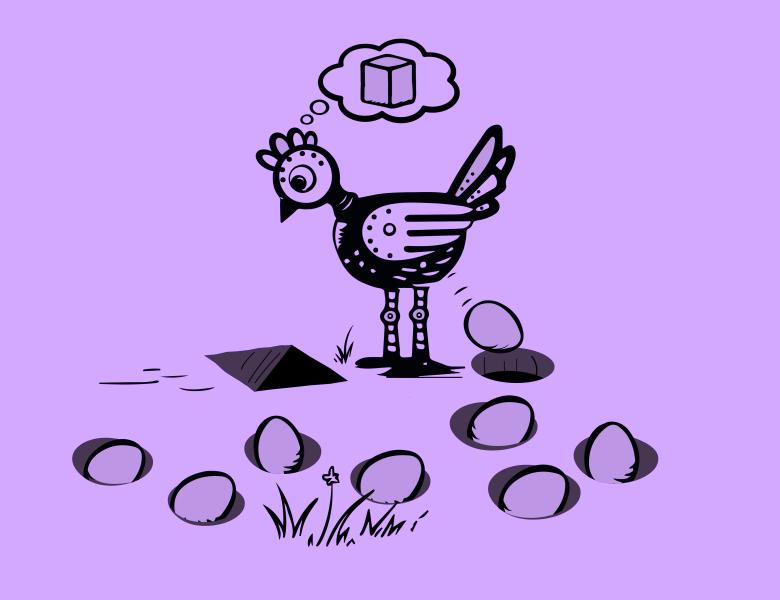
Abstract
The successes of large language models (LLMs) have transformed many domains, yet they do not always generalize well across all contexts, particularly in areas where social factors are involved. The talk examines LLM generalizations in social context from three perspectives: assessment, adaptation, and application. We first present a dynamic evaluation protocol based on directed acyclic graphs with varying complexity for assessing LLMs on many types of reasoning tasks. Then we explore how to adapt LLMs to be more socially generalizable by building culturally aware language technologies with an online-community driven knowledge base. Lastly, we discuss how to customize LLMs for social skill training in a variety of social contexts. Overall, we hope to provide insights into how LLM generalizes in social contexts and how to develop socially intelligent LLMs.