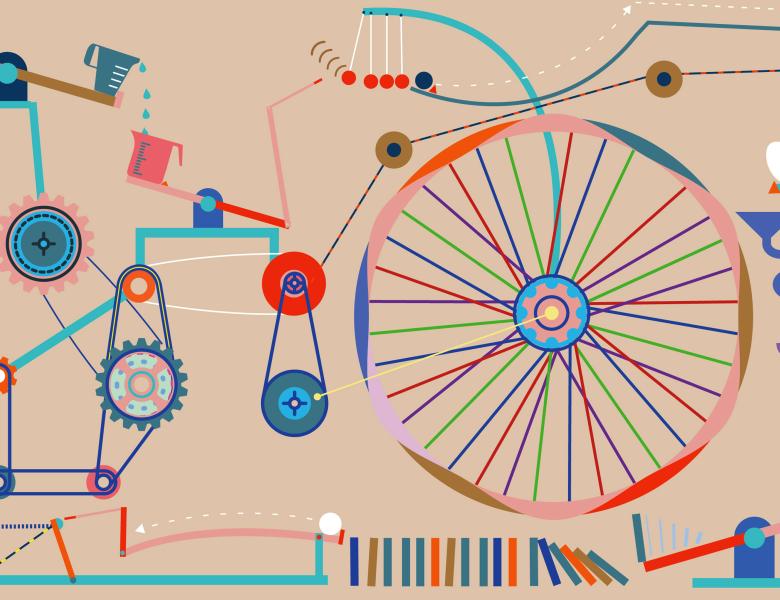
Abstract
In this talk, we will introduce a Collaborative Causal Discovery problem to model a common scenario in which we have multiple independent entities each with their own causal graph, and the goal is to simultaneously learn all these causal graphs. We study this problem without the causal sufficiency assumption, using Maximal Ancestral Graphs (MAG) to model the causal graphs, and assuming that we have the ability to actively perform independent single vertex (or atomic) interventions on the entities. If the M underlying (unknown) causal graphs of the entities satisfy a natural notion of clustering, we give algorithms that leverage this property, and recovers all the causal graphs using roughly logarithmic in M number of graphs atomic interventions per entity. We complement our results with a lower bound and discuss various extensions of our collaborative setting. Ideas from randomized algorithms play an important role in both our upper and lower bounds. Joint work with Raghavendra Addanki.