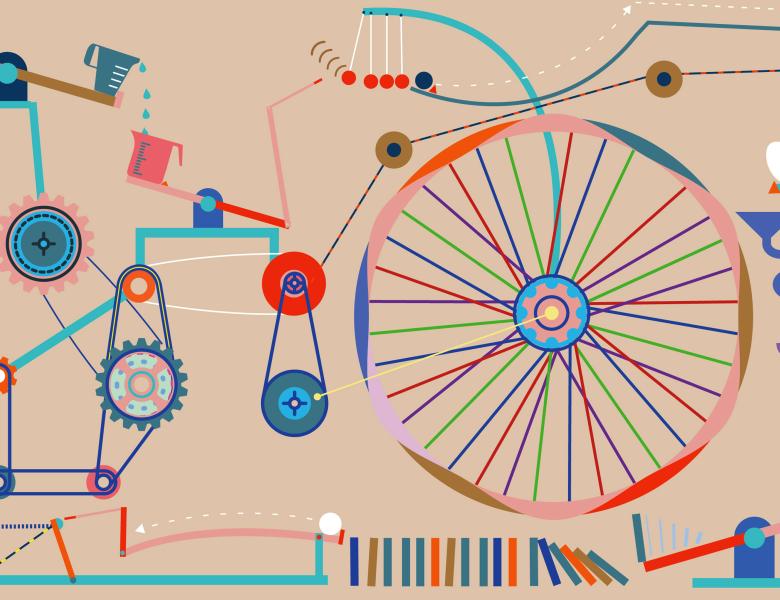
Abstract
We study the optimal sample complexity of neighbourhood selection in linear structural equation models, and compare this to conventional methods such as subset selection and the Lasso. We show by example that—even when the structure is unknown—the existence of underlying structure can reduce the sample complexity of neighbourhood selection. We further show how the rates depend on the effect of path cancellation, closely related to the notion of faithfulness, and that nonetheless the improvement persists even when there is path cancellation. Our results have implications for structure learning in graphical models, which often relies on neighbourhood selection as a subroutine.