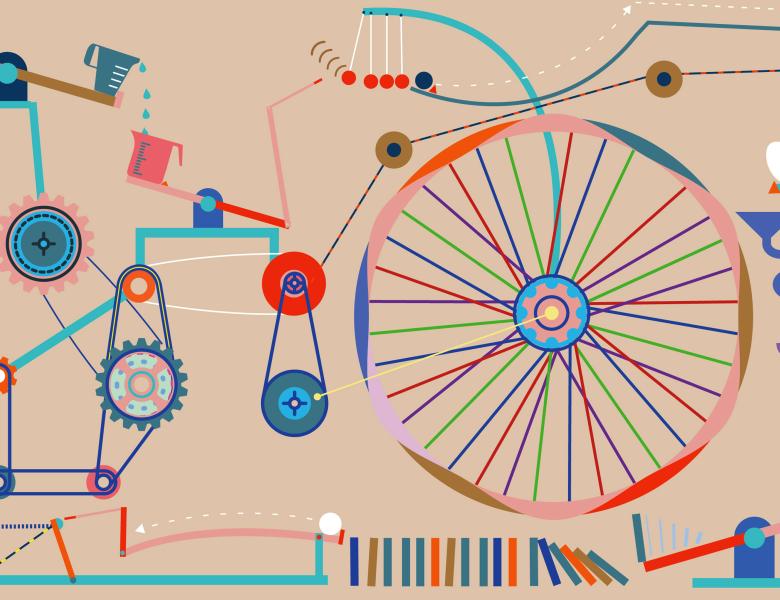
Abstract
Structural equation models use directed acyclic graphs to model the causal structure of a system. ``Causal discovery'' describes the problem of learning these structures from data. When data is gathered from multiple populations or environments, global confounding obscures the conditional independence properties that drive most causal discovery algorithms. We demonstrate that it is still possible to learn causal structures in the presence of global confounders with bounded cardinality. The feasibility of this problem is governed by a trade-off between the cardinality of the global confounder, the cardinality of the observed variables, and the sparsity of the causal structure.