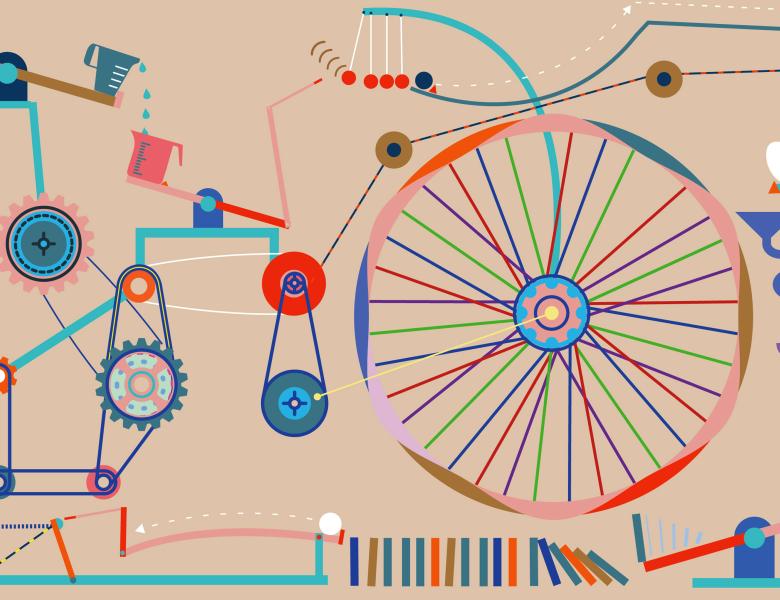
Abstract
The implication problem studies whether a set of conditional independence (CI) statements (antecedents) implies another CI (consequent), and has been extensively studied in the AI literature, under the assumption that all CIs hold exactly. A common example of implication is the well-known d-separation algorithm that infers conditional independence relations based on a ground set of CIs used to construct the graphical structure. However, many applications today need to consider CIs that hold only approximately. We define an approximate implication as a linear inequality between the degree of satisfaction of the antecedents and consequent, and we study the relaxation problem: when does an exact implication relax to an approximate implication? More precisely, what guarantee can we provide on the inferred CI when the set of CIs that entailed it hold only approximately? We use information theory to define the degree of satisfaction, and prove several results. In the general case, no such guarantee can be provided. We prove that such a guarantee exists for the set of CIs inferred in directed graphical models, making the d-separation algorithm a sound and complete system for inferring approximate CIs. We also prove an approximation guarantee for independence relations derived from marginal and saturated CIs. Next, we show how information-theoretic inequalities can be applied to the task of learning approximate decomposable models from observations.