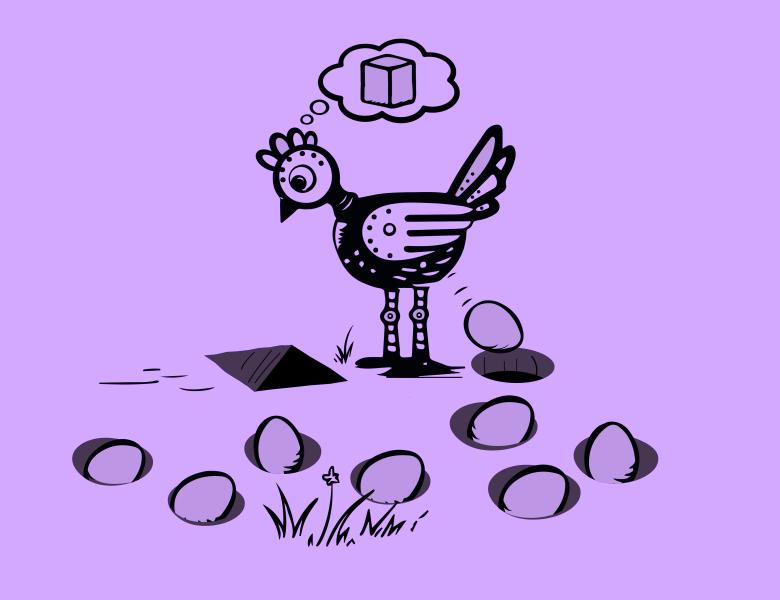
Abstract
I will describe empirical studies that explore very young children’s ability to use relational reasoning to generalize appropriately from small samples and to use analogical reasoning to predict the effect of novel visual transformations.These include increases in size and number, and rotations and reflections. In addition, I will describe a study comparing this ability to that of large multi-modal models.
In one study, toddlers appropriately generalized from a visual transformation on one type of object to transform another very different object. They learned how to use a novel “change machine” to make objects smaller or larger.In a second, preschoolers appropriately predicted the causal consequences of an even wider range of transformations. In a last study we propose a new benchmark for visual analogical reasoning in large models.While benchmarks exist for testing analogical reasoning in LMMs, they require advanced skills and omit basic visual analogies that even young children can make. Inspired by developmental psychology, we propose a new benchmark of 1,400 visual transformations of ev- everyday objects to test LMMs on visual analogical reasoning and compare them to children and adults. We structure the evaluation into three stages: identifying
what changed (e.g., color, number, etc.), how it changed (e.g., added one object),
and applying the rule to new scenarios. Our findings show that while models like
GPT-4V, LLaVA-1.5, and MANTIS identify the “what” effectively, they struggle
with quantifying the “how” and extrapolating this rule to new objects. In contrast, children and adults exhibit much stronger analogical reasoning at all three stages
Work with Eunice Yiu and Mariel Goddu.