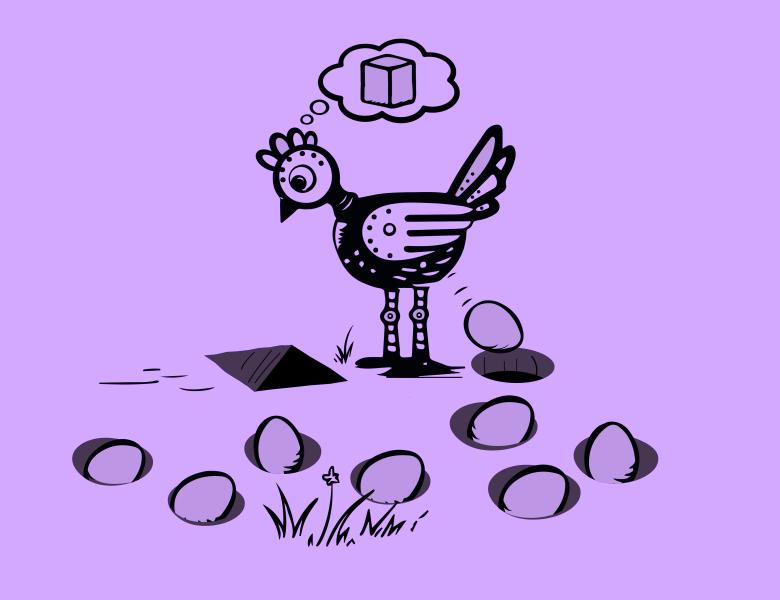
Abstract
Much research on fairness has focused on institutional decision-making tasks, such as resume screening. Meanwhile, hundreds of millions of people use chatbots like ChatGPT for very different purposes, ranging from resume writing and technical support to entertainment. We study “first-person fairness,” which means fairness toward the user who is interacting with a chatbot. The main challenge in analyzing first-person fairness is that chatbots generate open-ended text for a variety of tasks, hence existing fairness notions such as equalized odds do not necessarily apply. We present a methodology which can be applied to future chatbots as well as experiments demonstrating its effectiveness on ChatGPT. We find that post-training reinforcement learning significantly reduces harmful biases in ChatGPT.
This is joint work with Tyna Eloundou, Alex Beutel, David G. Robinson, Keren Gu-Lemberg, Anna-Luisa Brakman, Pamela Mishkin, Meghan Shah, Johannes Heidecke, and Lilian Weng.