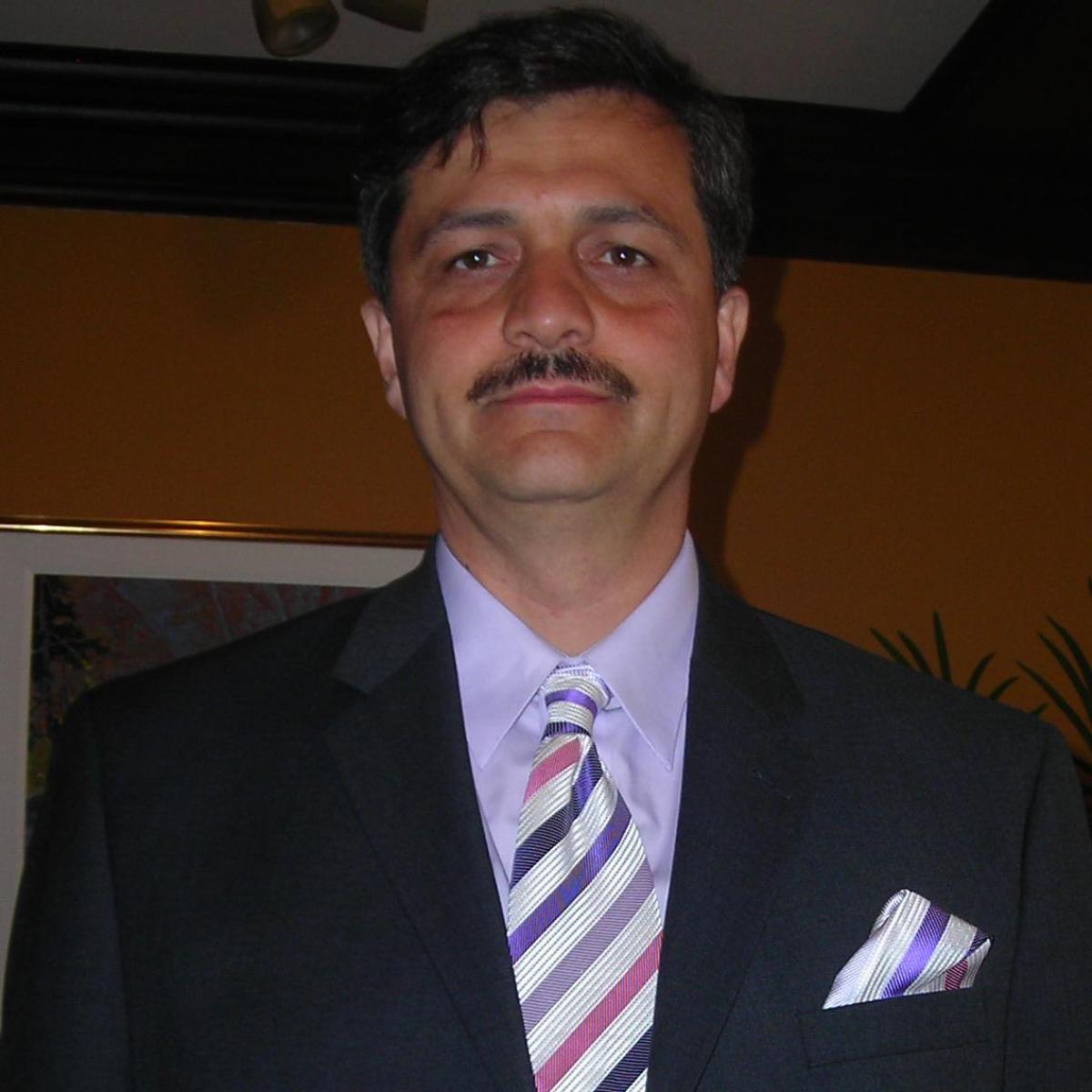
Farid Alizadeh
Farid Alizadeh is one of the originators of the field of semidefinite programming (SDP). He established the foundations of SDP, and applications to combinatorial optimization, as early as 1990. SDP has found numerous applications in a wide range of areas, including theoretical computer science, statistical learning theory, approximation algorithms, control theory, signal processing, statistics, shape optimization, and many other fields. In 1995, he became one among the first generation of researchers who received the NSF CAREER award. He is also the recipient of the 2014 INFORMS Optimization Society Farkas Prize. Alizadeh received his PhD from the Computer Science and Engineering Department at the University of Minnesota in 1991. His thesis advisor was Ben Rosen. He then spent two years at ICSI in Berkeley as a postdoctoral associate, working with Richard Karp on applications of combinatorial optimization in molecular biology, in particular gene mapping problems. He joined Rutgers University in 1994, first at RUTCOR and then in the Management Science and Information Systems Department of the Rutgers Business School. At Rutgers, he has worked on developing efficient algorithms for semidefinite and second order cone programming (SOCP) problems, applying SDP to statistical learning theory, especially shape constrained regression and density estimation. He has also worked on algebraic foundations of optimization, showing that essentially all algorithms developed for SDP can be extended to SOCP, and in general any symmetric cone. He demonstrated the SDP representability of sum-of-square cones arising from any formally real algebra. At present, Alizadeh is working on two broad areas. In one, he is attempting to extend simplex like algorithms (or first order methods) to SDP and SOCP, especially in a way that they can be used efficiently in branch and bound methods. He is also investigating learning problems where examples are augmented by rules (represented as regions in feature space).